Comparing ML performances of toxicity predictive models on a large cohort of breast cancer patients
Maria Giulia Ubeira Gabellini,
Italy
PD-0170
Abstract
Comparing ML performances of toxicity predictive models on a large cohort of breast cancer patients
Authors: Maria Giulia Ubeira Gabellini1, Martina Mori1, Alessandro Cicchetti1,2, Paola Mangili1, Gabriele Palazzo1, Andrei Fodor3, Antonella Del Vecchio1, Nadia Gisella Di Muzio4,5, Claudio Fiorino1
1IRCCS San Raffaele Scientific Institute, Medical Physics, Milan, Italy; 2Progetto prostata, Fondazione IRCCS Istituto Nazionale dei Tumori, Medical Physics, Milan, Italy; 3IRCCS San Raffaele Scientific Institute, Radiotherapy, Milan, Italy; 4 IRCCS San Raffaele Scientific Institute, Radiotherapy, Milan, Italy; 5 Vita-Salute San Raffaele University, Radiotherapy, Milan, Italy
Show Affiliations
Hide Affiliations
Purpose or Objective
Studies comparing the performances of machine learning (ML) methods in building predictive models of toxicity in RT are rare. Thanks to the availability of a large cohort (n=1323) of breast cancer patients homogeneously treated with tangential fields (Fodor et al. Clin Breast Cancer 2022), whose acute toxicity was prospectively scored, several ML approaches could be compared.
Material and Methods
The endpoint was RTOG G2/G3 acute toxicity, resulting in 209 and 1114 patients respectively with or without the event. The dataset, including 25 clinical, anatomical and dosimetric features, was split into 992 for the training and 331 for external test.
Several ML methods were considered: Logistic Regression (LR), Lasso, Elastic Net, k-Nearest Neighbors (KNN), Random Forest (RF), Support Vector Machines (SVM), Gaussian Naive Bayes (GNB) and Multi Layer Perceptron (MLP). First, we standardized the training dataset using the Robust Scaling, limiting the impact of outliers. The test dataset was consequently scaled accordingly. A One Hot Encoder on categorical features was applied and variables with high p-value at univariate LR were excluded; also features with Spearman ρ>0.8 were dropped. The Synthetic Minority Oversampling Technique was applied to create synthesized data of the minority to compensate class imbalance.
Finally, we run each model by applying a Bayesian search to maximize the chosen metrics (balanced accuracy and AUC) on a stratified K-Folds cross-validator sample. For each model, we derived the feature importance and complementary metric scoring for both training and test dataset. A Sequential Feature Selector was applied; we chose a parsimonious feature number for which AUC falls inside the minimum between 1% of the max metric value and its SD error. Procedures were implemented in Python v.3.7.9.
Results
As shown in Fig1, model’s performance were compared on training-test dataset over different metrics. The models are in ascending order starting from the one with less training-test discrepancy. Overall, the best models found were MLP and GNB. LR, more often used in literature, showed similar performance (as shown in Fig2, including features importance). KNN, RF and SVC tend instead to overfit.
The AUC of the test data is overall slightly above 0.6, f1 score on patients with toxicity is around 0.25, while it depends on model for patients without toxicity. MLP and GNB have best brier score, while LR is worse and more discrepant in test compared to training. The test slope derived from the calibration plot is low compared to the one expected and its R2 is quite different between training and test.
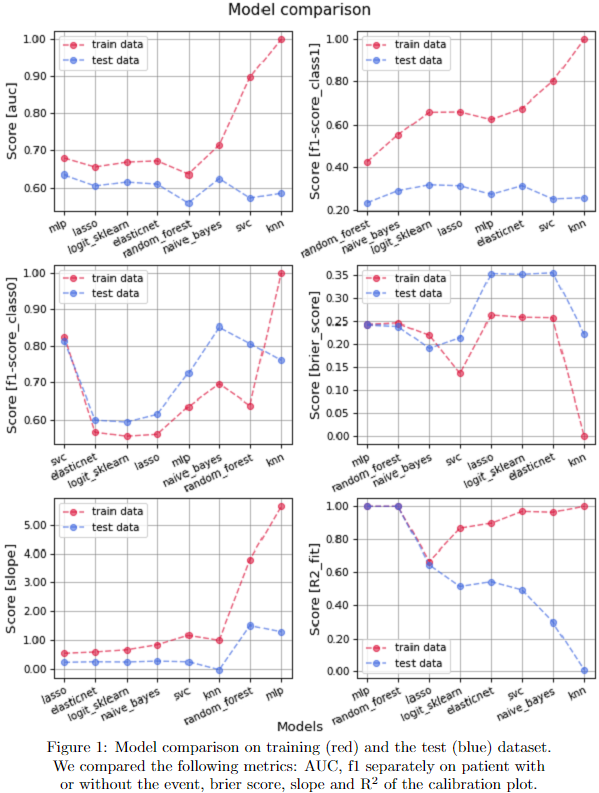
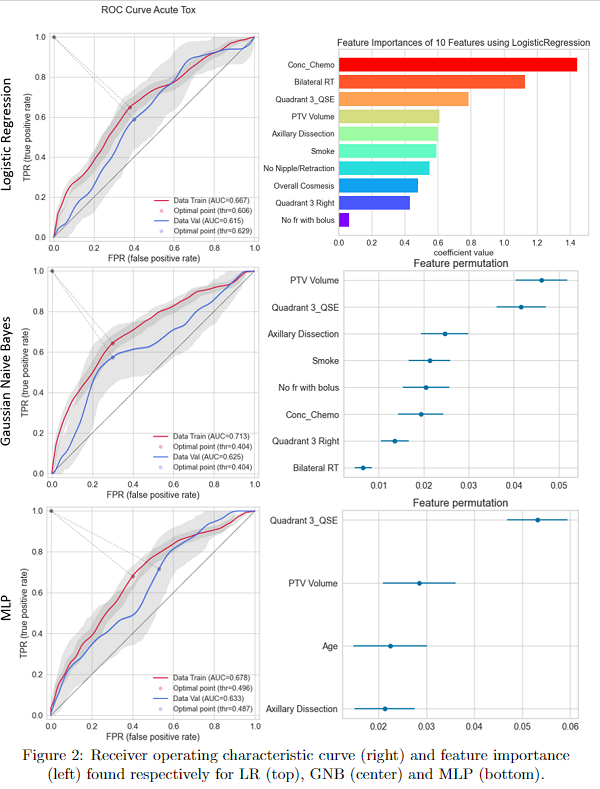
Conclusion
We set up a pipeline of pre-processing shared between different ML models and applied to a large cohort of patients to predict Acute Toxicity. As shown, no model performed the best for all metrics: more deep ML models have better performances, also if LR is not too different. For all of them we may quantify and see feature importance.
This study is supported by ERAPERMED-2020-110-JTC.