Predicted tumour probability maps improve deep learning outcome prediction in oropharyngeal cancer
Baoqiang Ma,
The Netherlands
PD-0167
Abstract
Predicted tumour probability maps improve deep learning outcome prediction in oropharyngeal cancer
Authors: Baoqiang Ma1, Alessia De Biase2,3, Jiapan Guo2,3, Lisanne V. van Dijk2, Johannes A. Langendijk2, Stefan Both2, Peter M.A. van Ooijen2,2, Nanna M. Sijtsema2
1University Medical Center Groningen, Department of Radiation Oncology, Groningen, The Netherlands; 2University Medical Centre Groningen, Department of Radiation Oncology, Groningen, The Netherlands; 3University Medical Centre Groningen, Data Science Centre in Health (DASH), Groningen, The Netherlands
Show Affiliations
Hide Affiliations
Purpose or Objective
Recently, deep learning (DL) algorithms showed to be promising in predicting outcomes such as distant metastasis-free survival (DMFS) and overall survival (OS) using pre-treatment imaging in head and neck cancer. Gross Tumour Volume of the primary tumour (GTVp) segmentation used as additional channel of DL input improved model performance. However, the binary segmentation mask of the GTVp directs the focus of the network to the defined tumour region only and uniformly. DL models trained for tumour segmentation have also been used to generate predicted tumour probability maps (PM) where each pixel value corresponds to the degree of certainty of that pixel to be classified as tumour. PM are less affected by inter-observer variability and include peritumoral regions with low probability values. The hypothesis we would like to test in this study is whether using PM instead of GTVp delineations as extra input channel for DL improves the predictive power of CT- and PET-based prediction models for oropharyngeal cancer (OPC) patients for local control (LC), regional control (RC), DMFS and OS .
Material and Methods
We included 399 OPC patients from our institute that were treated with (chemo)radiation between 2010 and 2021. For each patient CT and PET images and GTVp contours, used for radiotherapy treatment planning, were collected. We trained a previously developed 2.5D DL framework for tumour probability prediction by 5-fold cross validation using patients from 2019 onwards. Patients treated between 2010 and 2019 were used to train (168) and test (100) the DL model for outcome prediction with the previously mentioned 3D PM as one of the possible inputs. The predictive endpoints were LC, RC, DMFS and OS. The 3D ResNet18 was trained by 3-fold cross validation for each endpoint using different combinations of image modalities as input (see Figure 1). Then, the predictions of these three models were ensembled averaged in the test set. The C-index was used to evaluate the discriminative performance of the models.
Results
Table 1 shows that the models trained replacing the GTVp contours with the PM achieved highest C-indexes in LC (N° 5), RC (N° 3) and DMFS (N° 3) prediction, which may be due to the presence of low predicted probabilities in the lymph nodes and peritumoral regions in the PM. For OS, adding the PM (N° 3) or the GTVp (N° 4) to the PET resulted in comparable C-indexes Additionally, combing best DL models with clinical features further improved C-indexes to 0.61, 0.80 and 0.81 for RC, DMFS and OS prediction, respectively.
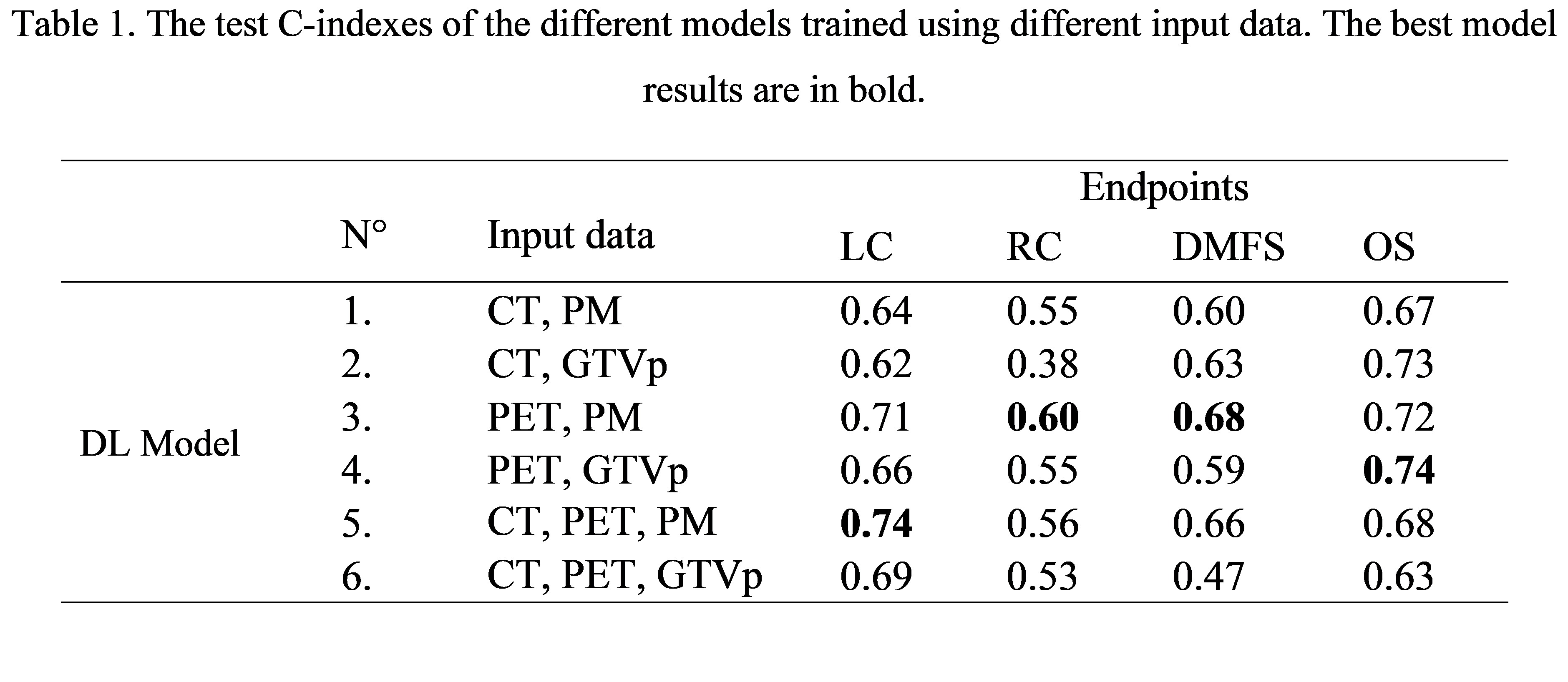
Conclusion
Adding predicted tumour probability maps instead of GTVp contours as an additional input channel for DL-based outcome prediction models improved the model performance for all outcome endpoints except for overall survival.