Do CBCT-based image synthesis models need to be machine-specific?
Chelsea Sargeant,
United Kingdom
PD-0665
Abstract
Do CBCT-based image synthesis models need to be machine-specific?
Authors: Chelsea Sargeant1, Jane Shortall1, Andrew Green2, Robert Chuter3,1, Alan McWilliam1
1The University of Manchester, Division of Cancer Sciences – Faculty of Biology, Medicine and Health , Manchester, United Kingdom; 2European Bioinformatics Institute, European Molecular Biology Laboratory, Hinxton, United Kingdom; 3The Christie NHS Foundation Trust, Christie Medical Physics and Engineering, Manchester, United Kingdom
Show Affiliations
Hide Affiliations
Purpose or Objective
Cycle-consistent generative adversarial networks (cycleGANs) are used to generate synthetic CTs (sCTs) from on-treatment CBCTs for radiotherapy treatment verification and enable daily plan adaptation. For optimal usability in the clinic, image synthesis models must be generalisable between systems and centres. Unlike CT machines, XVI systems do not give electron density information needed for a dose calculation, and CBCT image quality parameters are often machine-specific, despite the same machine model, software, and parameters functioning within manufacturer tolerance.
We investigate the impact of training with CBCT images from single vs. multiple systems for sCT generation to determine whether capturing such variability within training protocol leads to more generalisable models.
Material and Methods
Two cycleGANs were trained independently on two datasets consisting of 70 pelvic CBCT and planning CT (pCT) pairs. The 'clean' model was machine-specific; trained on 70 CBCT/pCT pairs from a single treatment machine, under consistent calibration settings, while the 'messy' model was trained on 70 CBCT/pCT pairs collected from five treatment machines in a single centre, with no attention paid to calibration dates.
For evaluation, the sCTs were compared against a corresponding deformed CT (dCT): the pCT deformably registered to the CBCT using ADMIRE v3.43.0 (Elekta AB). Both models were tested on two test datasets each consisting of an additional 25 CBCT/pCT pairs. The 'clean' test set was collected from the single treatment machine, and the 'messy' test set was from the five machines.
Global image similarity metrics; mean absolute error (MAE), root mean squared error (RMSE), structural similarity index (SSIM) and normalised cross-correlation (NCC), were calculated between sCTs and dCT within the body contour. A Wilcoxon signed-rank test was used to identify differences between the metrics.
Results
Results indicate that image synthesis models trained with multi-machine data outperform single-machine models on all test sets in terms of image similarity, shown by reduced MAE and RMSE, and increased SSIM (Figure 2A). Differences in CBCT machine and calibration within training data do not appear to hinder sCT image quality, instead offering increased generalisability. However, there is no significant difference between model performance (p≥0.068) as shown in Figure 2B i)-iv). This suggests that although initial results indicate that diverse training data improves sCT quality, dosimetric analysis is needed to indicate if this is significant.
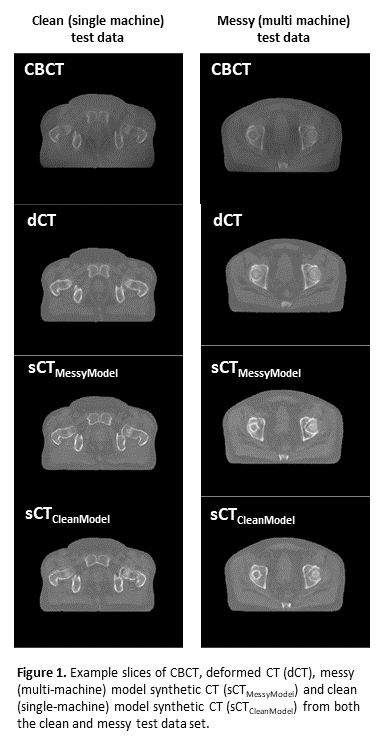
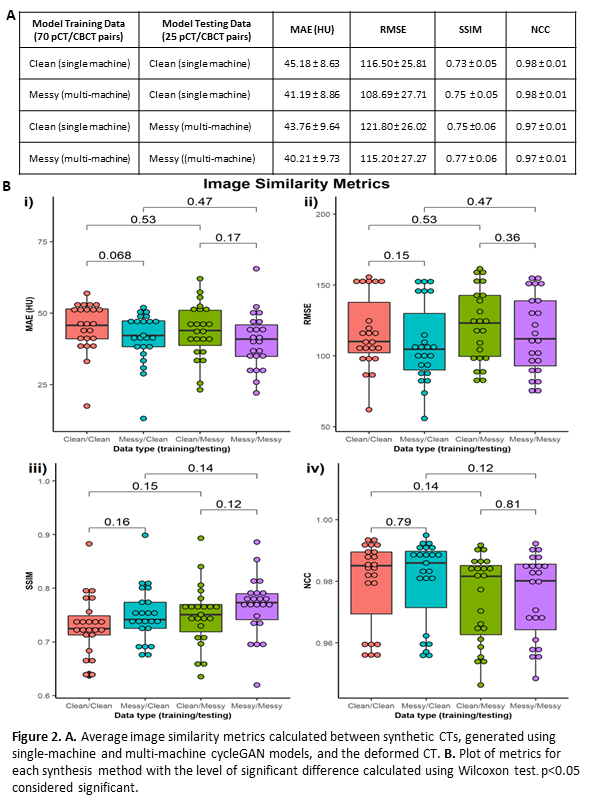
Conclusion
Initial results of image synthesis models trained on varied, multi-machine data outperform models trained on single-machine data. As an additional benefit, an sCT generation model, generalised to multiple machines, would be more easily deployed in the clinic. These results should be externally validated, and the effects on the clinical workflow e.g., dosimetric impact and contour propagation investigated.