Feasibility of markerless lung tumor tracking on radiographs with Retina U-Net
PO-1889
Abstract
Feasibility of markerless lung tumor tracking on radiographs with Retina U-Net
Authors: Lili Huang1,2, Christopher Kurz2, Philipp Freislederer2,3, Farkhad Manapov2, Stefanie Corradini2, Maximillian Niyazi2, Claus Belka2, Guillaume Landry2, Marco Riboldi1
1Ludwig-Maximilians-Universität München, Department of Medical Physics, Munich, Germany; 2University Hospital, LMU Munich, Department of Radiation Oncology, Munich, Germany; 3Brainlab AG, Brainlab, Munich, Germany
Show Affiliations
Hide Affiliations
Purpose or Objective
Lung tumors are subject to breathing motion and hence face motion-induced localization uncertainty. Conventionally, fiducial markers were employed to help localize tumor position in X-ray images, but the intrusive nature and the complication risk of marker implantation have led to explorations of markerless tracking approaches, especially using deep learning-based methods. However, few efforts have been made to systematically evaluate the applicability of these methods in individual cases. In this study, we adapted an object detection deep learning model for markerless lung tumor tracking by simultaneously predicting both a target bounding box and a segmentation mask. Furthermore, we compared the two types of predictions and proposed a patient-specific procedure to evaluate the feasibility of tracking individual cases with the model.
Material and Methods
Digitally reconstructed radiographs (DRRs, including projection of tumor segmentation) were generated from a public dataset comprising 875 CT scans and corresponding lung nodule annotations, and then used to train a Retina U-Net baseline model. A patient-specific refinement procedure was established using an independent cohort of 97 lung patients, among which 13 patients were reserved for fine tuning the automatic patient-specific training procedure and 37 were selected as test cases. The test set included only patients, on whose delineated planning CT (PCT)-DRR the baseline model predicted a target bounding box having an intersection over Union (IoU) with the ground-truth bounding box higher than 0.2. For each test set patient, the patient’s PCT-DRR and projected tumor delineation were used to refine the baseline model into a patient-specific model, which was then tested separately on the same patient’s 10-phase 4DCT-DRR (never used in training) to mimic the intrafraction motion during treatment. Prediction results of 2D segmentation masks were evaluated through the center of mass (COM) error and the dice similarity coefficient (DSC). Similarly, the center of box (COB) error and the DSC were used for the evaluation of bounding box detections.
Results
52% of the 97 patients had baseline model IoU above 0.2 on their PCT-DRR and were deemed trackable with this approach. On average, patient-specific models of the 37 testing patients showed a mean COM error of 2.6 mm (CT slice thickness was 3 mm), a mean segmentation DSC of 0.78, a mean COB error of 2.7 mm, and a mean box DSC of 0.83, with an inference time of 300 ms/frame. Patients with tumor motion amplitude above 5.9 mm (median amplitude of test set) showed slightly better results in all four metrics than those with lower tumor motion amplitude.
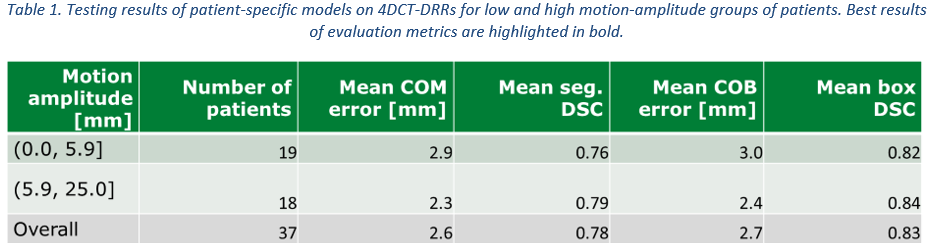
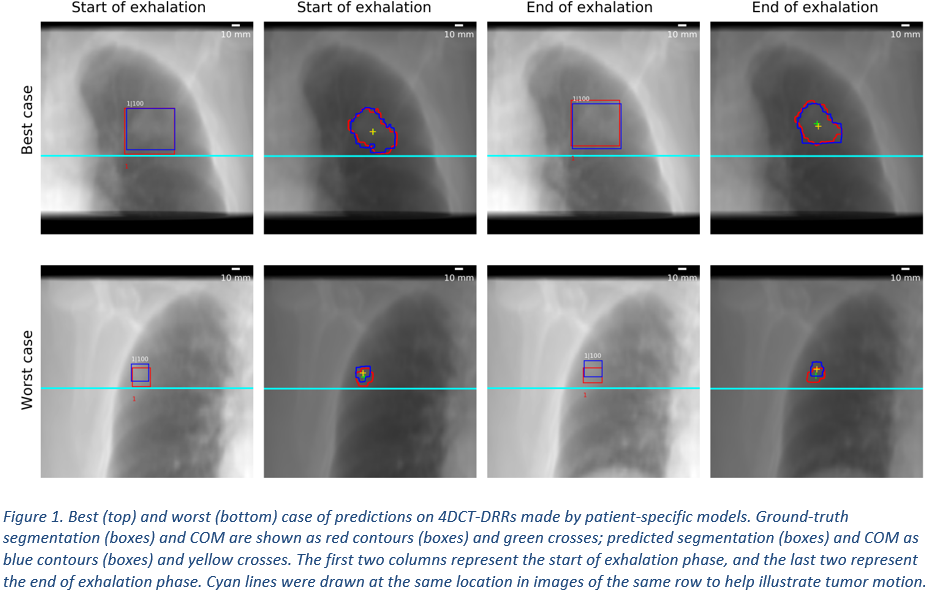
Conclusion
We implemented a patient-specific refinement approach using a pre-trained baseline model, which is suitable for real-time markerless lung tumor tracking. The implemented approach also provides quantitative criteria to select patients eligible for markerless tracking based on the PCT.