Clinical validation of AI-based CT auto-contouring for prostate cancer Radiotherapy
PO-1657
Abstract
Clinical validation of AI-based CT auto-contouring for prostate cancer Radiotherapy
Authors: Gabriele Palazzo1, Paola Mangili1, Chiara Deantoni2, Andrei Fodor2, Cesare Cozzarini2, Sara Broggi1, Antonella Del Vecchio1, Nadia G Di Muzio2, Claudio Fiorino1
1IRCCS San Raffaele Scientific Institute, Medical Physics, Milano, Italy; 2IRCCS San Raffaele Scientific Institute, Radiotherapy, Milano, Italy
Show Affiliations
Hide Affiliations
Purpose or Objective
To clinically validate an AI-based commercial system for auto-contouring for prostate cancer CT planning. Additional major aims were: a) quantifying the potential improvement of contouring consistency between different Radiation Oncologists; b) assessing the reduction of the time required for the segmentation of OARs and CTVs.
Material and Methods
Two Radiation Oncologists expert in segmentation of prostate cancer patients manually contoured CTVs (prostate and seminal vesicles) and OARs (rectum, bladder, femoral heads) of 20 patients, previously treated at our Institute, in the Eclipse TPS system (v 13). The delineated planning CTs were exported to the MIM system, with a dedicated module (MIM_protégéAITM) where we implemented a workflow performing automatic segmentation using AI. The automatically generated contours were sent back to Eclipse where observers reviewed a copy of the automatic contours and edited them to their needs. The operators were asked to evaluate the quality of the automated segmentation, with a 5-score scale (5: no editing necessary; 4: little editing; 3: moderate editing; 2: big editing; 1: almost complete manual replacement) and to report the time required for manual segmentation and for editing of the automatic contours. The agreement between manual vs automatic+edited contours was assessed through the DICE index. The same index was used to estimate Inter-observer variability for manual and automatic+edited segmentation.
Results
The system was able to generate all structures with a qualitatively high level. Femoral heads were not considered as the automatic system obtained clearly better results, likely due to the relatively low importance of this OARs, translating in a less accurate manual contouring. Median values of the clinical score were 4 for all structures with no scores below 3 and equal to 3 in a small fraction of patients for all structures (<20%). The reduction of time between manual and automatic+edited contours was large (mean: 24 vs 7 minute, p<0.01). Inter-observer variability ranged between 0.73 (seminal vesicles) and 0.93 (bladder), while the fast editing of the automatically generated structure sets resulted in an increase of DICE coefficients into the range 0.83 - 0.98 . These improvements, shown in Figure 1, were all significant (p<0.01). The intra-observer agreement between manual and automatic + edited structures resulted in DICE values similar to the ones of inter-observer variability, as shown in Fig 1. Of note, the average agreement between fully automated and manual contours was only slightly lower, with an average DICE value equal to 0.96 between automatic vs automatic+edited contours, confirming the prevalently little corrections made by both observers.
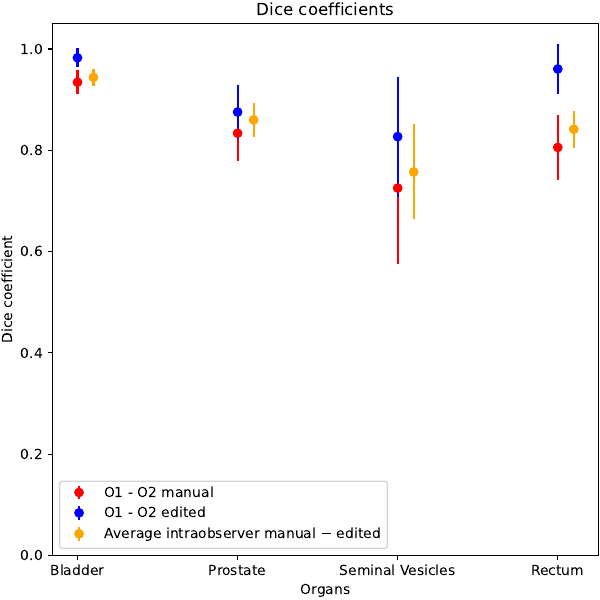
Conclusion
The available AI-based auto-contouring system can replace manual contour resulting in largely reduced time for segmentation and better consistency between operators.
The study is supported by AIRC grant IG 25951.