Does data curation matter in deep learning segmentation? Clinical vs edited GTVs in glioblastoma.
PO-1654
Abstract
Does data curation matter in deep learning segmentation? Clinical vs edited GTVs in glioblastoma.
Authors: Kim Hochreuter1,2, Jesper F. Kallehauge3,2, Jintao Ren1,2,4, Stine S. Korreman1,2,4, Slávka Lukacova2,4, Jasper Nijkamp1,2, Anouk K. Trip1
1Aarhus University Hospital, Danish Center for Particle Therapy, Aarhus, Denmark; 2Aarhus University, Department of Clinical Medicine, Aarhus, Denmark; 3Aarhus University Hospital, Aarhus, Denmark, Danish Center for Particle Therapy, Aarhus, Denmark; 4Aarhus University Hospital, Department of Oncology, Aarhus, Denmark
Show Affiliations
Hide Affiliations
Purpose or Objective
In postoperative chemoradiotherapy for glioblastoma (GBM) patients, the GTV is defined as contrast enhancement on T1w-MRI including the surgical cavity according to ESTRO guidelines. To automatically segment this GTV, deep learning (DL) models can be developed using clinical GTVs. However, clinical GTVs suffer from interobserver variation, which may impact DL-model performance.
The aim of this study was to compare performance of a DL-model based on clinical GTVs to a DL-model based on edited GTVs.
Material and Methods
259 consecutive patients with newly diagnosed GBM between 2012-2019 at a single center, each with a planning-MRI, and a clinical GTV following the ESTRO guideline, were included. For each patient, the clinical GTV was edited by a single independent radiation oncologist.
The cases were randomly split into train and test set (80:20), with stratification for extent of surgery (biopsy/limited/complete excision). We used nnU-net to train a model on clinical GTVs and similarly on edited GTVs, with contrast enchanced T1w-MRI as input. Both models were trained using 5-fold cross-validation, followed by ensemble-predictions (mean probability maps of the 5-folds) on the test set.
For evaluation surface Dice with a 2mm tolerance (sDSC) and Hausdorff Distance 95% (HD95) were reported in a cross-comparison using both models and delineation-sets (Fig. 1).
A. We first compared sDSC between models.
B. We compared sDSC of the clinical model evaluated on the clinical vs. the edited test set, to assess the impact of editing on model evaluation.
C. Similarly, we compared sDSC of the edited model evaluated on the clinical vs. the edited test set.
D. We then compared models again, while evaluating both models on the same edited test set.
For statistical comparison a paired Wilcoxon signed-rank test was used.
Results
Model:Evaluation | sDSC median (range)
| HD95 median (range) |
Clin:Clin | 0.91 (0.35-0.996)
| 3.0 (1.12-19.34)
|
Edit:Edit | 0.98 (0.79-0.9999)
| 1.66 (1.0-44.11)
|
Clin:Edit | 0.94 (0.66-0.993)
| 2.26 (1.26-37.55)
|
Edit:Clin | 0.90 (0.37-0.995)
| 3.18 (1.12-43.66)
|
The Edit model evaluated on the Edit test set (Edit:Edit), outperformed all other model test combinations (Fig. 1ACD & 2, table). We only present p-values for sDSC comparisons, as the conclusions for HD95 are identical.
Interestingly, the sDSC results significantly improved when the clinical model was evaluated on the edited test set instead of the clinical (Clin:Clin vs. Clin:Edit) (Fig. 1B). Altogether showing that both the clinical and edited model predictions better resembled the edited GTVs in the test set. Moreover, when evaluating with the clinical test set, there was no significant difference between the models (Fig 2, Clin:Clin vs. Edit:Clin p=.36).
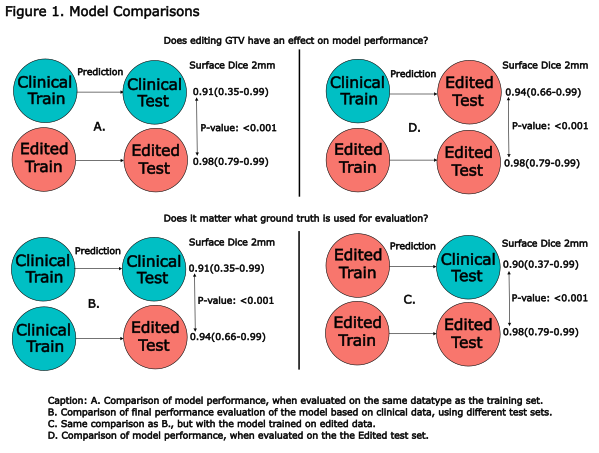
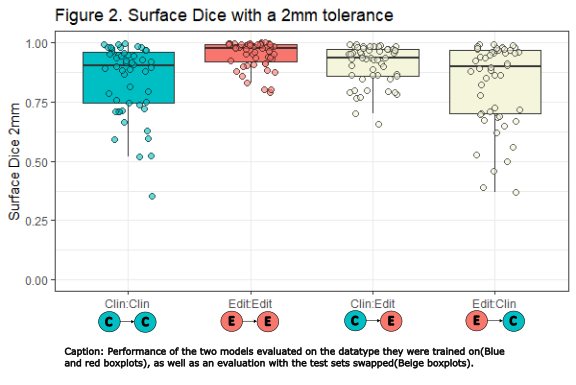
Conclusion
Data curation had a significant impact on model performance, and on the evaluation of model performance. The model based on curated data had a substantially higher precision and accuracy than the model based on clinical data. Our results emphasize the importance of data curation in DL.