Dosimetric Evaluation of MR-based Deep Learning Automatic Contouring in the Pelvis
PO-1628
Abstract
Dosimetric Evaluation of MR-based Deep Learning Automatic Contouring in the Pelvis
Authors: Jonathan Wyatt1, Laszlo Rusko2, Rachel Pearson3, Bernadett Kolozsvári2, Borbala Deák-Karancsi2, Vanda Czipczer2, Zsofia Karancsi4, Eszter Ruff2, BlankaHelga Irmai2, BulsciPeter Tass2, Katalin Hideghéty2, Steven Petit5, Marta Capala5, Florian Wiesinger6, Ross Maxwell1, Hazel McCallum1
1Newcastle University, Institute of Translational and Clinical Research, Newcastle upon Tyne, United Kingdom; 2GE Healthcare, Computer Science, Budapest, Hungary; 3Newcastle upon Tyne Hospitals NHS Foundation Trust, Northern Centre for Cancer Care, Newcastle upon Tyne, United Kingdom; 4Ge Healthcare, Computer Science, Budapest, Hungary; 5Erasmus MC Cancer Institute, Department of Radiotherapy, Rotterdam, The Netherlands; 6GE Healthcare, Magnetic Resonance Physics, Munich, Germany
Show Affiliations
Hide Affiliations
Purpose or Objective
Manual contouring of OARs is critical for radiotherapy but is time-consuming and prone to inter-observer variability. MR imaging has reduced contouring variability due to its superb soft-tissue contrast. This may also facilitate automatic contouring algorithms, which would further improve consistency and efficiency, especially within MR-only workflows. The aim of this study was to develop a MR-based Deep Learning automatic contouring algorithm for pelvic OARs and evaluate it dosimetrically.
Material and Methods
A set of 2D and 3D convolutional neural networks were trained separately to contour bladder, bowel bag, femoral heads, rectum, penile bulb, prostate, prostate & seminal vesicles and urethra. The model was trained on T2-weighted MR images from 88 patients (13F, 75M) from a 1.5T (n=49) or 3T (n=39) scanner using manual contours by medical students trained by clinical oncologists.
The algorithm was evaluated on 3T T2-weighted MR images from 20 patients (6F, 14M) treated for prostate (n=10), rectal (n=4) or anal (n=6) cancers. A VMAT plan was optimised on CT using the clinical PTV and automatic OAR contours to deliver 60 Gy in 20 fractions (prostate), 50.4/45/25 Gy in 28/25/5 fractions (rectal) or 53.2/50.4 Gy in 28 fractions (anal). Doses to the automatic and manual contours were determined and differences at clinically relevant dose constraints calculated. A Consultant Clinical Oncologist rated each contour for clinical acceptability on a 5-point scale (1-Delete, 2-Major, 3-Intermediate, 4-Minor, 5-Accept). Automatic and manual contours were compared using Dice.
Results
Automatic contours were successfully generated for every organ in all patients except urethra (failed for two patients) and penile bulb (one patient). Mean DVH differences between automatic and manual contours were < 1.0 Gy/percentage point for all OARs except bowel bag (table 1). This is within the 1 Gy dose difference reported for manual inter-observer variability.
Table 1 Mean ± standard error dose differences, Dice scores and clinical ratings.
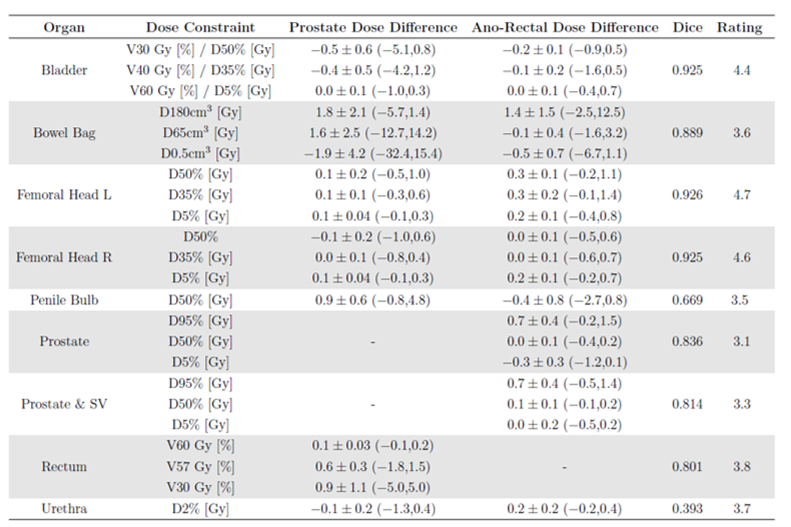
The bowel bag dose differences were substantially larger for the prostate plans than the ano-rectal plans. This was due to over-segmentation in the inferior direction, which resulted in very large dose differences for the prostate plans due to the steep dose gradient in this region (figure 1). This suggests the dosimetric evaluation is highly dependent on the treatment site.
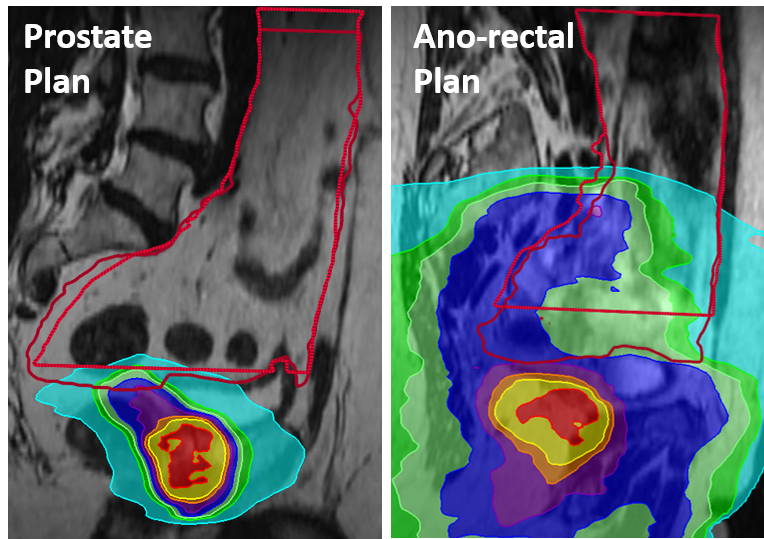
Figure 1 Example dose distributions and bowel bag discrepancies for manual (light red) and automatic (dark red) contours.
Conclusion
A MR-based Deep Learning automatic OAR contouring algorithm has been successfully developed for pelvic OARs. This has been dosimetrically evaluated with mean dose differences at clinically relevant DVH points being < 1 Gy/percentage point for bladder, femoral heads, rectum, penile bulb, prostate, prostate & seminal vesicles and urethra contours. Therefore the algorithm is sufficiently accurate for clinical use for these organs for prostate and ano-rectal radiotherapy plans.