Multicentric evaluation of a machine learning model to streamline the RT patient-specific QA process
PO-1617
Abstract
Multicentric evaluation of a machine learning model to streamline the RT patient-specific QA process
Authors: Nicola Lambri1,2, Victor Hernandez3, Jordi Sáez4, Marco Pelizzoli1, Sara Parabicoli1, Andrea Bresolin1, Damiano Dei1,2, Ciro Franzese1,2, Pasqualina Gallo1, Francesco La Fauci1, Francesca Lobefalo1, Lucia Paganini1, Giacomo Reggiori1,2, Stefano Tomatis1, Daniele Loiacono5, Marta Scorsetti1,2, Pietro Mancosu1
1IRCCS Humanitas Research Hospital, Radiotherapy and Radiosurgery Department, Milan, Italy; 2Humanitas University, Department of Biomedical Sciences, Milan, Italy; 3Hospital Universitari Sant Joan de Reus, Department of Medical Physics, Tarragona, Spain; 4Hospital Clínic de Barcelona, Department of Radiation Oncology, Barcelona, Spain; 5Politecnico di Milano, Dipartimento di Elettronica, Informazione e Bioingegneria, Milan, Italy
Show Affiliations
Hide Affiliations
Purpose or Objective
Patient-specific quality assurance (PSQA) is an important step of intensity modulated plan verification to ensure that treatment plans can be delivered as intended. The time and effort required to perform measurement-based PSQA constitutes a substantial workload that could slow down the radiotherapy process and delay the start of clinical treatments. In this study, a machine learning (ML) tree-based ensemble model to predict the gamma passing rate (GPR) was developed, and its applicability in three independent Institutions was evaluated.
Material and Methods
5622 VMAT plans from multiple treatment sites were selected from the internal database of Institution 1. After a thorough data cleaning procedure, ~2% of candidate plans were discarded. XGBoost, a tree-based ensemble ML model, was trained on 5522 VMAT plans using 19 input features (10 plan complexity metrics and 9 plan parameters). The GPR analyses were performed automatically on acquired images using the criteria 3%/1 mm (global normalization with absolute dose, 10% threshold) and 95% action limit. To examine the sensitivity of the model to the density of data points above 95% GPR, where more than 80% of the GPRs resided, the training set was randomly undersampled. The ratio of the minority class (i.e., GPR <95%) over the majority class (i.e., GPR >=95%) was increased from 20% of the complete training set, to 40%, 60%, 80%, and 100%. Then, for each undersampling level, a new regression model was trained. Models performance was evaluated on an out-of-sample test set of Institution 1 and on two independent sets of measurements collected at Institution 2 and Institution 3. The mean absolute error (MAE), absolute error statistics, as well as the models’ sensitivity and specificity, were computed.
Results
Figure 1 shows the distribution of the residuals (i.e., the difference between measurements and predictions) for each Institution for the model trained on all available training data (20% class balance). Small positive median values were observed (0.95%, 1.66%, and 3.42%). Thus, the model’s predictions were, on average, close to the real values and, in most cases, tended to slightly underestimate the experimental GPR, providing a conservative estimation. Table 1 reports the evaluation metrics of the regression models for each Institution. In general, an increase in class balance was associated with a degradation in the MAE and specificity, whereas the models’ sensitivity improved. The model trained on all available training data (20% class balance) achieved the lowest MAE of 2.33%, 2.54%, and 3.91% on the three Institutions, with a specificity of 0.90, 0.90 and 0.68, and a sensitivity of 0.61, 0.25, and 0.55, respectively.
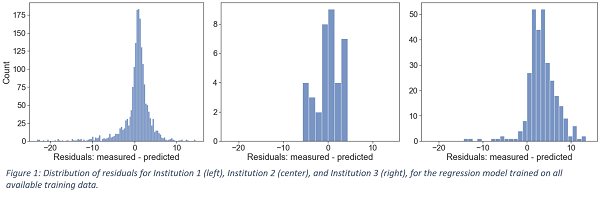
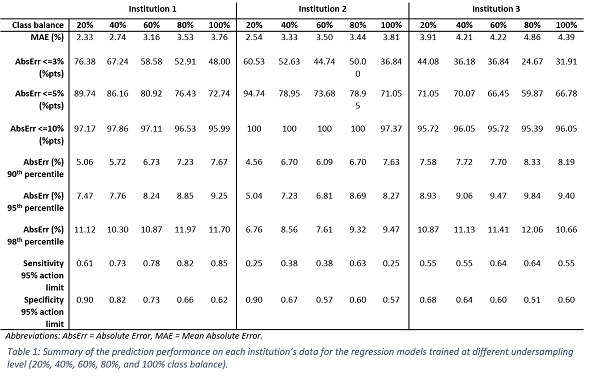
Conclusion
Our results indicate that ML models can be integrated into clinical practice to streamline the radiotherapy workflow, but they should be centre-specific or thoroughly verified within centres before clinical use.