Alternative DL segmentation approaches for clinical partially-labeled HN data: transformers or Unet?
PD-0326
Abstract
Alternative DL segmentation approaches for clinical partially-labeled HN data: transformers or Unet?
Authors: Lucía Cubero Gutiérrez1, Lucía Cubero Gutiérrez2, Joël Castelli2, Renaud de Crevoisier2, Oscar Acosta2, Javier Pascau1,3
1Universidad Carlos III de Madrid, Departamento de Bioingeniería, Madrid, Spain; 2Université Rennes, CLCC Eugène Marquis, Inserm, LTSI - UMR 1099, Rennes, France; 3Hospital Gregorio Marañón, Instituto de Investigación Sanitaria Gregorio Marañón, Madrid, Spain
Show Affiliations
Hide Affiliations
Purpose or Objective
Deep learning (DL) has recently demonstrated efficiency and robustness for automatic segmentation of organs at risk (OAR) in radiotherapy (RT). Head and neck (HN) RT treatment planning could particularly benefit from this tool due to the large amount of OAR present in the region and the difficulties for manual segmentation driven by the varying shapes of some organs, anatomical deformations induced by the tumor, and low contrast between tissues. These issues also diminish the efficacy of DL models, which are usually trained and evaluated in single-center curated datasets. This study aimed to compare the performance of two state-of-the-art DL networks for HN OAR segmentation trained with a partially-labeled clinical database, where each patient has different OAR manually contoured.
Material and Methods
The study included 225 partially-labeled CT images from HN cancer patients with locally advanced carcinoma of the oropharynx, a condition that often hampers OAR contouring. These data were used to train a two-step workflow to segment 11 OAR. First, single-class OAR-specific networks based on 3D U-Net were trained to generate pseudo-contours for the CTs with missing labels, obtaining a fully-segmented training image set. Then, a multiclass network was trained with 5-fold cross-validation to segment the 11 OAR simultaneously, exploiting the anatomical relationships between the individual structures. In this step, we compared the performance of two state-of-the-art DL algorithms: nnU-Net, a self-configuring fully-convolutional neural network, and SwinUNETR, a model introducing vision transformers with self-attention mechanisms to the task of delineation. These two models have shown competitive results in semantic segmentation but, to our knowledge, have never been implemented to segment partially-labeled clinical HN datasets. Both algorithms were evaluated on 44 fully-labeled CT images excluded from training by measuring the Dice Score Coefficient (DSC) and Average Surface Distance (ASD).
Results
Figure 1 depicts the evaluation metrics of both DL models on the test set. nnU-Net achieved slightly more accurate results for almost every OAR. Nonetheless, the differences in performance were shallow, and both networks achieved very accurate results for all OAR except the lips, submandibular glands, and larynx (DSC < 75%). Each fold of nnU-Net was trained in 23 hours, whereas SwinUNETR required 40 hours per fold.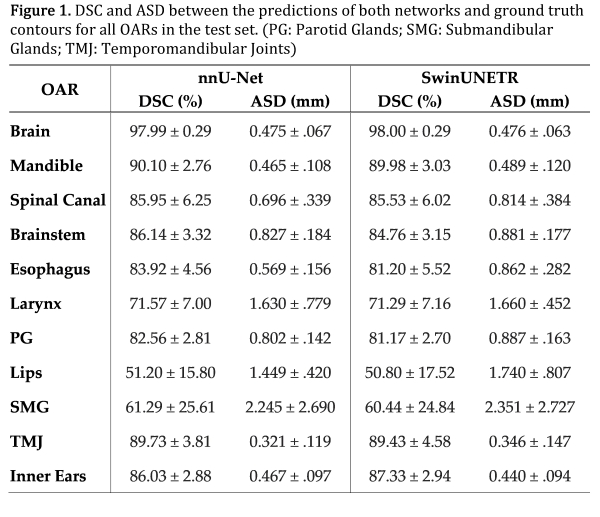
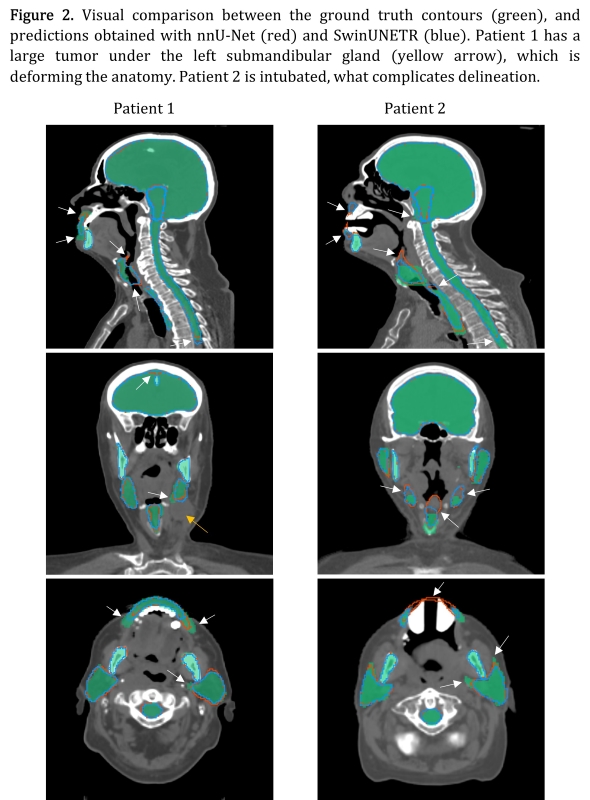
Conclusion
We compared the performance of two state-of-the-art DL algorithms, which have reported outstanding semantic segmentation results, when trained with a partially-labeled clinical database. The predicted contours were very accurate with both models for almost all OAR. The underperformance on three structures was probably driven by the presence of large tumors and other external devices, such as gastro and nasopharyngeal tubes, deforming the anatomy and hindering segmentation (Figure 2). Overall, nnU-Net showed better results in terms of accuracy and computational requirements.