Unsupervised generation of synthetic CT from CBCT using 3D GAN in head and neck cancer radiotherapy
PO-1677
Abstract
Unsupervised generation of synthetic CT from CBCT using 3D GAN in head and neck cancer radiotherapy
Authors: Cédric Hémon1, Blanche Texier1, Pauline Lekieffre1, Emma Collot1, Caroline Lafond1, Anaïs Barateau1, Renaud De Crevoisier1, Jean-Claude Nunes1
1LTSI, INSERM, UMR 1099, Univ Rennes 1, CHU Rennes, CLCC Eugène Marquis, Rennes, France
Show Affiliations
Hide Affiliations
Purpose or Objective
Several approaches to generate synthetic CT (sCT) from CBCT have been developed for dose replanning in ART. Recently, deep learning methods are making constant progress in sCT generation but unsupervised learning has been less investigated. The purpose of this study is to evaluate the use of different perceptual loss implementations to help the generation in unsupervised learning.
Material and Methods
For this study, 43 patients with locally advanced oropharyngeal carcinomas treated with external beam radiation therapy were selected. Among our patients, each received a planning CT scan and 14 patients received a weekly CT scan. All CT were acquired on a BigBore scanner (Philips). CBCTs were acquired with an XVI (Elekta) on a VERSAHD linac (Elekta) for a time close to that of the CTs.
For the study, each sCT is generated by a 3D Generative Adversarial Network (GAN) composed of two adversarial networks (the generator and the discriminator). As a generator, we used a custom 6-block residual network between 2 down-sampling blocks and 2 up-sampling blocks, and the discriminator is a 70*70*70 PatchGAN network.
In unsupervised training, training is often challenged by learning problems such as instability and non-convergence of training. To reduce the occurrence of its problems, generator has been guided by using a perceptual loss.
We proposed 4 different models all based on the custom GAN with different loss function: VGG Perceptual loss, ConvNext Perceptual loss and ConvNext Perceptual loss +Gradient loss.
To generate a sCT, we used the principle of perceptual loss. The sCT is generated from the addition of the content loss of the input (CBCT) and the style loss of a CT. A new implementation of ConvNext-based perceptual loss is proposed to reduce the memory space used to compute the VGG perceptual loss. The layers used for the computation of style and content loss will be chosen after a detailed analysis by a feature map inversion.
The model was trained using the AdamW optimizer with a learning rate of 0.0001 for 200 epochs. Each model was trained under the identical hyper-parameter settings, while varying the definition of loss function.
The sCTs were compared to the original CTs based on a per-voxel comparison with mean absolute error (MAE) in Hounsfield units (HU).
Results
Figure 1 shows the sCT generated from the CBCT of an average test patient in terms of MAE with the different methods.
Figure 2 shows the MAE for the external body on the test patients from the different methods. Significantly lower values (p-value <= 0.0001, given by a signed-rank Wilcoxon test between the ConvNext method and the VGG method) were obtained when using the ConvNext-based perceptual loss.
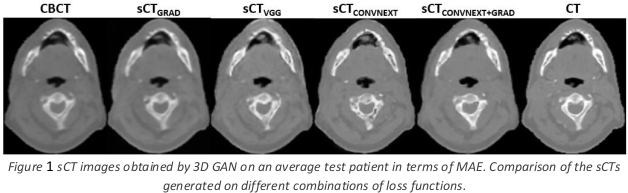
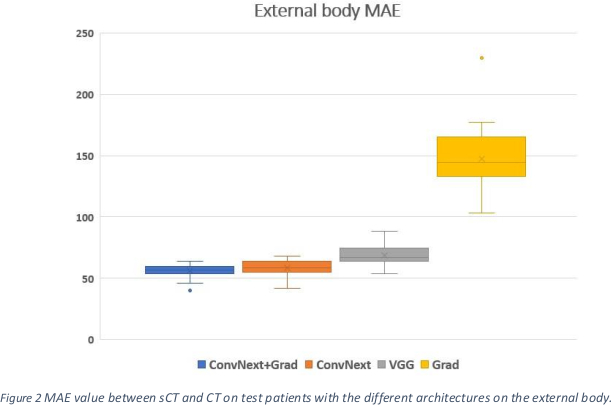
Conclusion
The use of ConvNext-based perceptual loss results in a significant visual and quantitative improvement in unsupervised sCT generation.
Our work shows: (1) The feasibility of training with non-registered data using perceptual loss (2) an alternative to VGG for the perceptual loss must continue to be studied.