3D patch cycle-GAN-based MR-to-CT synthesis from monocenter and multicenter training
PO-1671
Abstract
3D patch cycle-GAN-based MR-to-CT synthesis from monocenter and multicenter training
Authors: Pauline Lekieffre1, Emma Collot1, Blanche Texier1, Cédric Hémon1, Safaa Tahri1, Hilda Chourak1,2, Igor Bessieres3, Peter Greer2, Jason Dowling2, Anaïs Barateau1, Caroline Lafond1, Renaud de Crevoisier1, Jean-Claude Nunes1
1LTSI, INSERM, UMR 1099, Univ Rennes 1, CHU Rennes, CLCC Eugène Marquis, Rennes, France; 2CSIRO Australian e-Health Research Centre, Herston, Queensland, Australia; 3Centre Georges-François Leclerc, Departement of Medical Physics, Dijon, France
Show Affiliations
Hide Affiliations
Purpose or Objective
To increase the accuracy of cancer radiation therapy, many MR-to-CT deep-learning methods have been developed, including the 3D cycle-GAN architecture. The aim of this study is to compare a monocentric and a multicentric training of this architecture to generalize the model for use in all cancer centers.
Material and Methods
For this study, prostate CT and MR images were acquired in treatment position for 79 patients from two centers. The first center is composed of 39 patients. CT scans have been acquired with a GE LightSpeedRT large-bore scanner or a Toshiba Aquilion. MR acquisitions, using 3D T2-weighted SPACE sequences, were performed with a 3T Siemens Skyra MRI scanner. The other 40 patients are from a second center where the CT scans were acquired with a GE Medical Systems LightSpeed RT16 (2.5 mm slice thickness) and the 3DT2/T1-weighted SPACE MRI with a 0.35T ViewRay Inc. Linac MRIdian. The cycle Generative Adversarial Network (cycleGAN) combines two GAN in opposition and has a ResNet 9 block as a generator and a 70*70*70 PatchGAN network as discriminator. The images were distributed into training cohorts of 20 patients and test cohorts of 10 patients. A cohort of 10 patients from each center was used for training in the multicenter study. All the images in the train were cut into four patches that overlapped on the half and data augmentation through rotations is applied during training with a maximum angle of 30°. The loss functions used are the L1 norm as cycle loss with a weight of 30, a binary cross-entropy (bce) as adversarial and a L1 norm as identity, both with a weight of 1. The learning rate is worth 0.0001 and there are 100 epochs. Synthetic CTs (sCTs) were compared to the original CTs by a voxel-wise comparison on body and bones with the mean absolute error (MAE) in Hounsfield units (HU), mean error (ME) in HU too and peak signal to noise ratio (PSNR) in dB. The Wilcoxon test was used to compare the results obtained with the different trainings. Significant differences were considered for p-value < 0.05.
Results
Table 1 shows MAE, ME and PSNR for body and bone contours for monocenter and multicenter training cohort. Figure 1 presents a comparison of the sCT obtained with the different tests. There is a presence of artifacts during one train from center 2 followed by a test from center 1. The Wilcoxon test shows a significant difference between monocentric studies and those with a different training and testing center. On the contrary, the p-value is high when comparing the monocenter study with the study that has a composite train of data from both centers.
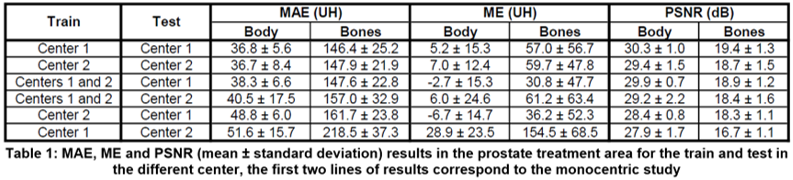
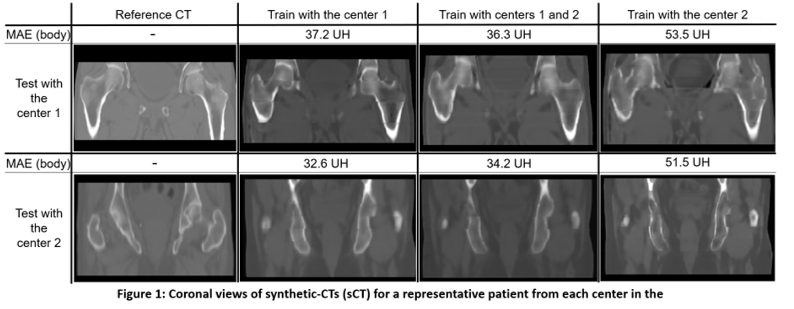
Conclusion
This comparison between the monocenter and multicenter trainings for two different centers showed that there was no significant difference in terms of outcomes and images when the learning cohort contains data from both centers.