An automated strategy to evaluate the structure consistency of CBCT-to-CT synthesis
Catarina Veiga,
United Kingdom
PO-1689
Abstract
An automated strategy to evaluate the structure consistency of CBCT-to-CT synthesis
Authors: Adam Szmul1, Sabrina Taylor1, Isabel Moreira1, Pei Lim2, Jessica Cantwell2, Derek D'Souza2, Syed Moinuddin2, Mark Gaze2, Jennifer Gains3, Catarina Veiga1
1University College London, Medical Physics and Biomedical Engineering, London, United Kingdom; 2University College London Hospitals NHS Foundation Trust, Radiotherapy, London, United Kingdom; 3University College London Hospitals NHS Foundation Trust,, Radiotherapy, London, United Kingdom
Show Affiliations
Hide Affiliations
Purpose or Objective
Synthetic CTs (synCTs) that match the anatomy of daily CBCTs have important applications in adaptive radiotherapy workflows. It is challenging to validate and compare the quality of synCT images generated with different popular methodologies, such as deformable image registration (DIR) or artificial intelligence (AI). In this work we propose an automated tissue segmentation method to assess the structural consistency between matched CBCT and synCTs.
Material and Methods
We propose to evaluate the structural similarity between matched synCTs and CBCTs using segmentations of key tissue types within the body: soft tissue, skeleton, gastrointestinal (GI) air, and lungs. We automated the segmentation of these tissues on CT/CBCT using an AI-based method. CT/CBCT scans and semi-automatic (ground-truth) segmentations of all tissue types from 63 patients aged 2 to 22 historically irradiated in the thoracic-abdominal-pelvic region were used. We trained a patch-based 3D UNet (96×96×48) with 4 downsampling/upsampling stages (16, 32, 64, 128, 256 channels at each of the levels respectively) with two residual blocs at each resolution level. The training dataset (N=50) consisted of CBCT/CTs aiming for the network to perform well on both CBCT and CT-like images. The network was then used to segment an independent dataset (N=13): planning CT, daily CBCTs and two synCT images matched to each daily CBCT (Fig 1). One synCT was generated by deformably registering the CT to CBCT (synCT DIR); the other was generated using an in-house cycleGAN network that converted CBCT to CT (synCT AI). The structural similarity was evaluated using Dice Similarity Coefficient (DSC), the Hausdorff Distance (HD) and mean pixel intensity (HU).
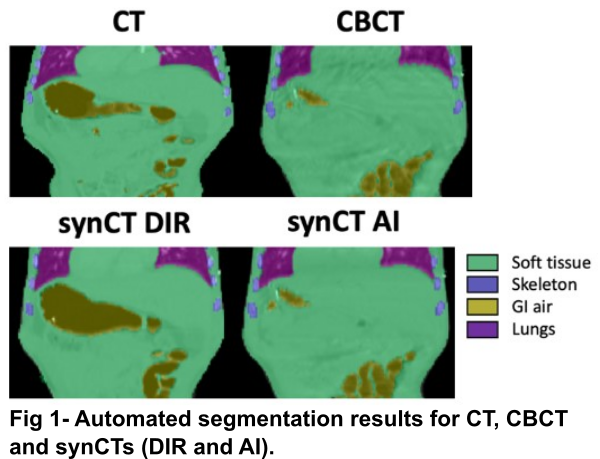
Results
The network segmented well real CT and CBCT images with DSC ranging 0.89 – 0.98 for all tissue types (Tab 1, “Validation”). The automatic contours of GI gas separated individual gas pockets more commonly than ground-truth segmentations. Contrast enhanced vessels were sometimes mislabelled as skeleton. The network allowed us to quantitatively compare the quality of different synCTs, which were compared against ground-truth segmentations on CBCT (Tab 1, “Application”). In general, synCT AI performed the best in terms of structure consistency. While both methods performed similarly in the lungs (HD={3.5, 3.5} mm for {DIR, AI}), the AI method was advantageous to DIR in GI gas regions (HD={24.0, 6.1} mm for {DIR, AI}). This indicates the need for additional strategies to account for non-deformable anatomical change when using DIR. Note that GI gas was segmented correctly as suggested by the similarity in HUs between CT and synCTs (HU={-805, -762, -759} for {CT, DIR, AI}).
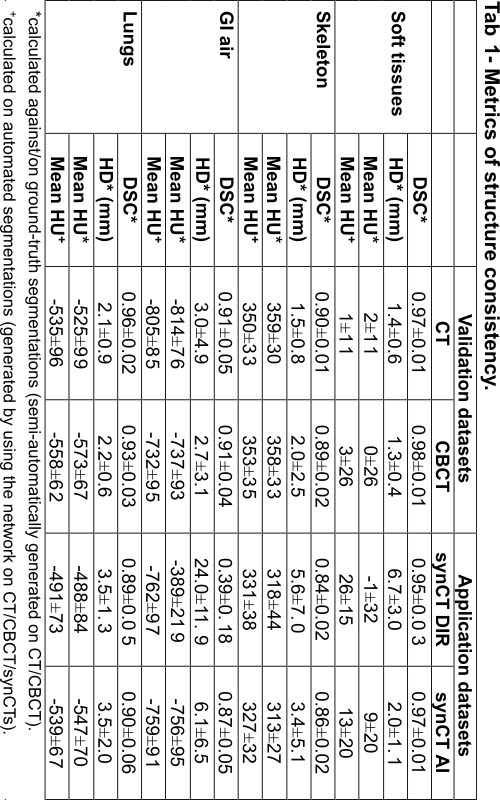
Conclusion
The proposed automated tissue segmentation method is well-suited to evaluate the structure consistency of synCTs. It can used to quantitatively compare different methodologies and identify their limitations. It also has potential application in automatic quality assurance of synCTs in clinical workflows.