Reconstruction of the mid-position image using NiftyReg and verification of the mid-position.
Helen Grimes,
United Kingdom
PO-1685
Abstract
Reconstruction of the mid-position image using NiftyReg and verification of the mid-position.
Authors: Alice Carlin1, Helen Grimes2, Jamie R McClelland3, Bjoern Eiben4, Callum Gillies5
1Barts Health NHS Trust, Radiotherapy Physics, London, United Kingdom; 2University College London NHS Foundation Trust, Radiotherapy Physics, London, United Kingdom; 3University College London Centre for Medical Image Computing, Department of Medical Physics and Biomedical Engineering, London, United Kingdom; 4Institute of Cancer Research and Royal Marsden NHS Foundation Trust, Joint Department of Physics, London, United Kingdom; 5University College London NHS Foundation Trust, Radiotherapy Physics Services, London, United Kingdom
Show Affiliations
Hide Affiliations
Purpose or Objective
Respiratory motion is a large contributor to uncertainty for lung radiotherapy patients, partly due to artefacts in the CT images. One of the most common approaches for managing motion is to use a 4DCT data set to determine the CTV-ITV margin from the tumour motion over all 4DCT phase images. This can lead to large PTVs for moving tumours which in reality spend little time at the extremities. Another method is to generate a mid-position (MidP) image showing the tumour at its time-weighted mean position, and accounting for the motion in the CTV-PTV margin recipe. This enables improved image quality and a reduction of the required CTV-PTV margins for subjects whose tumours move. This project aims to develop and validate a process for generating the MidP image using open-source software in a cohort of lung cancer patients.
Material and Methods
Fig 1 shows the processes used to develop the MidP image. The mean and median MidP images were generated using NiftyReg deformable image registration software from 4DCT data sets for a cohort of 12 lung cancer patients. Matlab was required for the median averaging. Image quality was compared between the mean and median MidP images, Ave image and individual phase images. The accuracy of the method was determined by acquiring 4DCT images of a geometric ISIS phantom using sinusoidal and patient representative breathing traces, then comparing the reconstructed mid-position with the expected mid-position from the trace data. This was measured as the difference between the centre of mass of the moving sphere in the MIP and MidP profiles to determine the shift of the mid-position from the geometrical mid position.
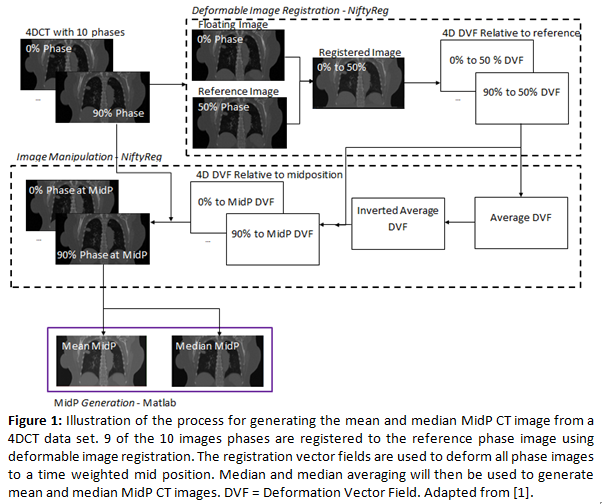
Results
A method for generating the MidP image was developed using NiftyReg. The median MidP image was more robust than the mean MidP image when considering the effect of mis-registration errors in the MidP image. Fig 2 shows a comparison between the Ave image and reconstructed mean and median MidP images for a single patient. When comparing the MidP image to the Ave image, image quality was improved when comparing the visualisation of structures within the lung. When considering the images of the ISIS phantom, the SNR from the different density inserts was higher on the MidP images when compared to the phase images. The reconstructed MidP image was accurate to within 0.1 mm and 0.65 mm of the calculated mid-position for the sinusoidal trace and representative breathing trace, respectively.
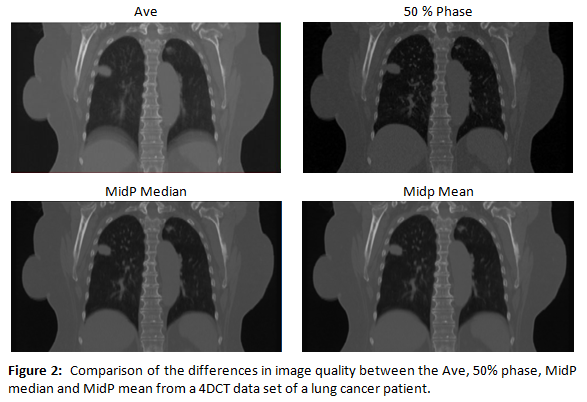
Conclusion
This project has developed an automated method for generating the MidP image using NiftyReg software for deformable image registration. The results show that image quality is improved when using a MidP dataset compared to Ave dataset or phase image, and the median averaging approach is more robust to mis-registration artefacts. The findings also validate the accuracy of the reconstruction of the mid-position to within 0.65 mm.
[1] Wolthaus, J W H (2009). Four-dimensional imaging in radiotherapy for lung cancer patients. Nederlands Kanker Instituut.