Towards tumor tracking by using deep learning to correlate lung contour deformation feature and skin
PO-1905
Abstract
Towards tumor tracking by using deep learning to correlate lung contour deformation feature and skin
Authors: Jie Zhang1, Yajuan Wang1
1The Cancer Hospital of the University of Chinese Academy of Sciences (Zhejiang Cancer Hospital), Department of Radiation Physics, Hangzhou, China
Show Affiliations
Hide Affiliations
Purpose or Objective
To propose a cascade ensemble model to track tumor motion by detecting respiration-induced changing skin. To ensure applicability to various patients, the lung contour deformation feature (LCDF) is an intermedia in the proposed model to correlate the internal target with changing skin.
Material and Methods
The proposed cascade ensemble model consists of three sub-models. The first one generates skin deformation feature (SDF) by inputting reference skin and changing one. The second one generates LCDF based on SDF. The third one estimates the tumor displacement by correlating LCDF and the reference tumor position. The first sub-model is the encoder of a U-net. The U-net is a network to register the deformable skin to its reference one. Similarly, LCDF is derived from the encoder of a U-net which aims to match changing lung contour to its reference one. The sub-models were trained on 117 sample pairs from 11 sets of four-dimension computed tomography(4D-CT) and were tested on 20 sample pairs from 4 sets of 4D-CT. Each 4D-CT embraced at least 4 sets of three-dimension (3D) CT which corresponded to different respiratory phases in a breathing cycle. The tumor location, lung contour and skin extracted from the 3D-CT at 0th phase served as the reference sample in the ensemble model. The 3D CT images at the remaining phases were the deformable samples, and constituted sample pairs with their reference samples. These scans came from patients with tumor in lung. The evaluation was performed on all sample pairs using average ± standard deviation of the tracking error, and was performed on each test set using root-mean-squared error (RMSE) as defined in the following formula:
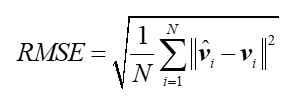
where vi was the ture location of tumor at ith phase. ^ represented the estimated value. N was the number of test phases for one patient. || || meant Euclidean distance.
Results
The 20 test samples showed tracking error of 3.10±1.45mm (average ± standard deviation). The RMSEs of the 4 test sets were 3.40±1.54mm (range: 1.10~5.09mm). Their corresponding target motion ranges were 9.68~11.77mm.
Conclusion
The proposed cascade ensemble model shows promising in accurate tumor motion tracking without patient-specific training before treatment.