Synthetic 4DCT generation from 4DMRI of the abdominal site in Carbon Ion Radiotherapy
PO-1869
Abstract
Synthetic 4DCT generation from 4DMRI of the abdominal site in Carbon Ion Radiotherapy
Authors: Anestis Nakas1, Maksym Hladchuk1, Giovanni Parrella1, Alessandro Vai2, Silvia Molinelli2, Mario Ciocca2, Andrea Pella3, Amelia Barcellini4, Viviana Vitolo4, Sara Imparato4, Ester Orlandi4, Guido Baroni1,3, Chiara Paganelli1
1Politecnico di Milano, Bioengineering, Milan, Italy; 2National Center for Oncological Hadrontherapy (CNAO), Medical Physics, Pavia, Italy; 3National Center for Oncological Hadrontherapy (CNAO), Bioengineering Unit, Pavia, Italy; 4National Center for Oncological Hadrontherapy (CNAO), Clinical Unit, Pavia, Italy
Show Affiliations
Hide Affiliations
Purpose or Objective
To demonstrate the ability of our Deep Learning network to generate abdominal synthetic 4DCTs (s4DCTs) from 4DMRI data of patients undergoing Carbon Ion Radiotherapy (CIRT) to complement treatment planning and off-line adaptation strategies.
Material and Methods
MRI acquisitions were performed on 24 patients with abdominal tumors treated with CIRT at the National Center for Oncological Hadrontherapy (CNAO, Italy) in correspondence of the planning (or re-evaluation) 4DCT. The MRI protocol consisted in T1-weighted (T1-w) 3D MRI acquired at the end exhale respiratory phase and dynamic 4D T2-wighted (T2-w) MRI on a limited field of view (FOV) due to spatial-temporal trade-off. T1-w 3D MRI guarantees accurate visualization of patient anatomy, whereas dynamic acquisitions allowed to reconstruct T2-w 4DMRI and obtain respiratory motion information. Virtual 4DT1-w MRI were then generated by applying the deformation vector fields (DVFs), obtained through Deformable Image Registration (DIR) among T2-w 4DMRI respiratory phases, to the T1-w 3DMRI acquired at end exhale. The limited FOV was extended according to Meschini et al., Med Phys, 2020. We previously trained a modified version of the ‘pix2pix’ architecture (Isola et al., 2017, Meschini et al., ESTRO 2021) on 39 pairs of T1-w 3D MRI and correspondent CT data at the exhale phase to generate 3D synthetic CT (sCT). Here, we tested the previously trained network on virtual T1-w 4DMRI data of three patients to generate s4DCTs. Multiple metrics were used to evaluate the generated s4DCTs such as the Mean Absolute Error (MAE), Root Mean Squared Error (RMSE), Normalized Cross Correlation (NCC), Structural Similarity Index (SSI) and Peak Signal to Noise Ratio (PSNR).
Results
A visual representation of the generated s4DCT and virtual 4DT1s with respect to the planning 4DCT for four different respiratory phases can be seen in Fig. 1. The quantitative results are summarized in Table 1. The accuracy of the used test set was in agreement with other studies concerning sCT generation.
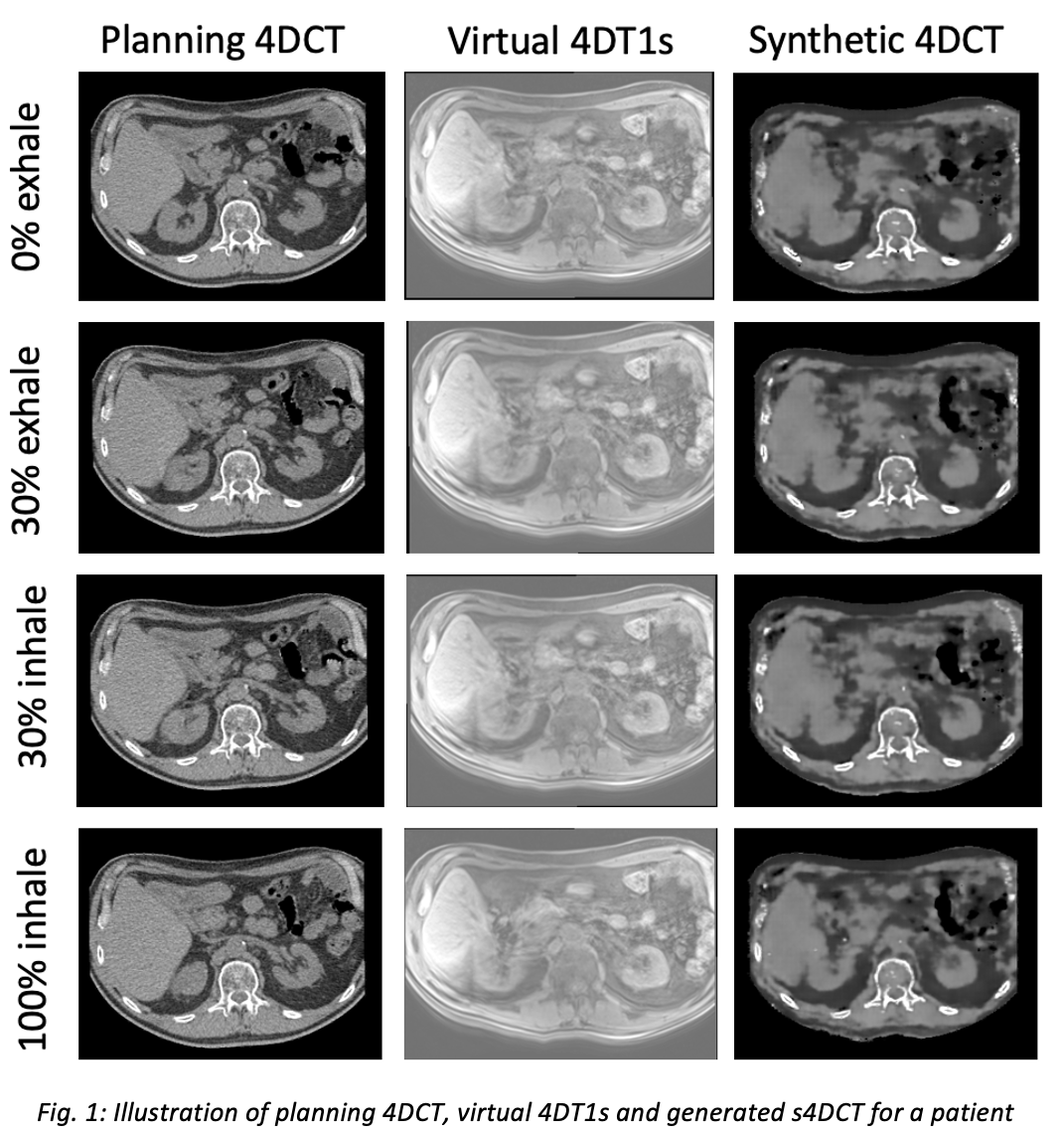
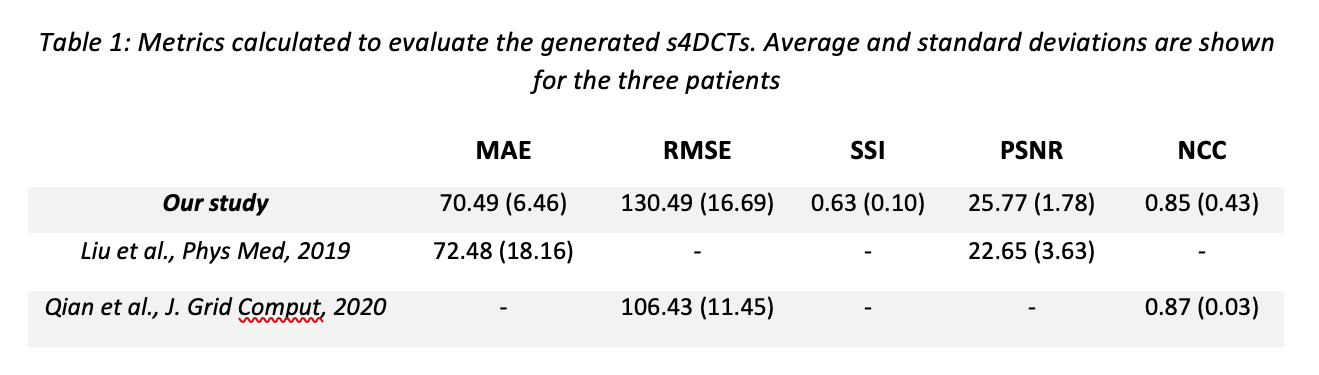
Conclusion
Our results demonstrate the good performance of our network in generating s4DCT of the abdominal site. These could be used to support treatment planning and off-line adaptation within the CIRT workflow of abdominal tumors. In the future, our network’s performance will be tested with larger patient datasets and a dosimetric analysis will be performed.