Self-learning GAN based synthetic CT generation: unlocking CBCT-based adaptive radiotherapy
MO-0389
Abstract
Self-learning GAN based synthetic CT generation: unlocking CBCT-based adaptive radiotherapy
Authors: Thais Roque1, Ayoub Oumani2, Edouard Delasalles3, Nikos Paragios4, Pascal Fenoglietto5
1TheraPanacea, Clinical affairs, Paris, France; 2TheraPanacea, AI engineering, Paris, France; 3TheraPanacea, Physics, Paris, France; 4Centrale Supelec, University of Paris-Saclay, Deparment of Mathematics, Gif-sur-Yvette, France; 5L'Institut du Cancer de Montpellier, Department of Radiation Oncology, Montpellier, France
Show Affiliations
Hide Affiliations
Purpose or Objective
Cone-beam CT (CBCT) is an essential component of treatment delivery in radiation therapy. To date, its main usage is primarily devoted to patient positioning due to limited quality, resolution, and field of view. Harnessing and using CBCT beyond patient positioning could contribute to the effective implementation of adaptive treatment at scale. This would require improving substantially the quality of signal and augmenting the field of view such that organ at risk annotation, full scale dose simulation and replanning can be performed. In this study an artificial intelligence-based pseudo CTs (AICT) is proposed and clinically evaluated to overcome these challenges and potentially unlock the full potential of CBCT for adaptive radiotherapy for pelvic cancer care.
Material and Methods
For the case of pelvis CBCT treatment adaptation, transfer learning was applied to an automatic synthetic-CT (sCT) generation tool from unpaired pelvis CTS and CBCTs that uses ensembled self-supervised GANs. The training cohort included over 600 planning CTs with corresponding CBCTs. An independent, retrospective cohort of 20 prostate cancer patients treated at two European cancer care excellence centres were selected for this evaluation. Planning CTs were deformably registered to the CBCTs for each patient to account for changes in the patient body and positioning. Treatment plans were optimized on the warped CT (wCT) and recalculated on the sCTs for image and dosimetric evaluation. For the analysis, wCTs and sCTs were compared based a) DVH-parameters (D2%, D50%, D95%, D98% and Dmean) for the PTV, and b) dose distributions compared with global gamma criteria (2%/2mm and 3%/3mm).
Results
Table 1 shows comparative results of differences in DVH between the dose calculated on the sCTs with extended FoV generated from the CBCTs and the dose calculated on the warped CTs. The differences in DVH are represented by the median relative difference with the minimum (min) and the maximum (max) values for the seven DVH indicators: Dmean, Dmax, D98, D95, D50, D5 and D2 for PTV. The highest and lowest difference were observed for the D98% (0.552%) and D5% (0.153%) parameters, respectively. The differences in gamma pass rate, represented by the median values, were 99.23%, 99.55% and 99.89% and 99.88%, 99.97% and 100% for 10%, 20% and 40% cut off dose for 2%/2mm and 3%/3mm, respectively.
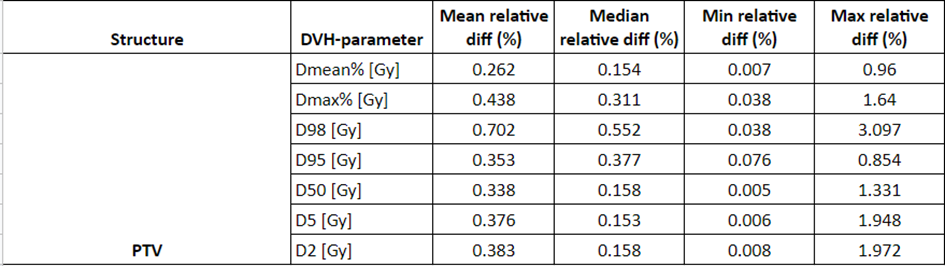
Table 1: Overall dosimetric results comparing synthetic CTs and warped CTs
Conclusion
In this work, we have demonstrated the feasibility of using artificial intelligence to transform CBCT images to high resolution CT. The present evaluation demonstrated the non-inferiority of using CBCT-based synthetic CT for treatment planning in terms of dosimetric measures. Next steps will include the integration of this work in the context of an effective implementation of an adaptive workflow from OARs annotation to full scale dose simulation and replanning.