predicting radiation pneumonitis based on retraining a deep learning feature extraction model
Zhixiang Wang,
The Netherlands
PO-1784
Abstract
predicting radiation pneumonitis based on retraining a deep learning feature extraction model
Authors: Zhixiang Wang1, Zhen Zhang1, Alberto Traverso1, Andre Dekker1
1Maastricht University, Department of Radiation Oncology (Maastro), Maastricht, The Netherlands
Show Affiliations
Hide Affiliations
Purpose or Objective
Radiation pneumonitis (RP) is a common adverse effect of thoracic radiotherapy. At present, some researchers use the pre-trained convolutional neural network(CNN) to extract deep learning (DL) features which have been proved that it can achieve better performance than the hand-craft feature extraction method. Although using a pre-trained model is easier to migrate to other tasks, only a model that has been retrained according to a specific data set can be best applied to a specific task. Till now, no one has trained a model for RP tasks. In this way, we re-train the pre-train model to extract DL features to make the extracted features suit the RP task.
Material and Methods
CT images and dose files were obtained on 349 patients diagnosed with lung cancer who received radical radiotherapy at Tianjin cancer hospital between 2013 to 2019. Compared with the pre-trained model trained on the UCF101 video dataset, we used a linear DL classifier with the cross-entropy loss function to re-train the model.
Two experiments were used to prove the features created by re-trained CNN are more suitable for this task than the pre-trained CNN. First, the Principal Component Analysis (PCA) method was used to show the distribution of the re-trained features and pre-trained features. Second, we separate features into training and testing set as 7:3 rate and built a classification model based on logistic regression trained and tested by retrained features and pre-trained features, respectively, then AUC (under the curve) was used to compare the classification performance of the features.
Results
First, the PCA method shows that the re-trained features have significant differences between positive and negative samples than pre-trained features. Second, compare with the classification model trained and tested by pre-trained features with an AUC score was 0.58, the re-trained features have a higher score of 0.65.
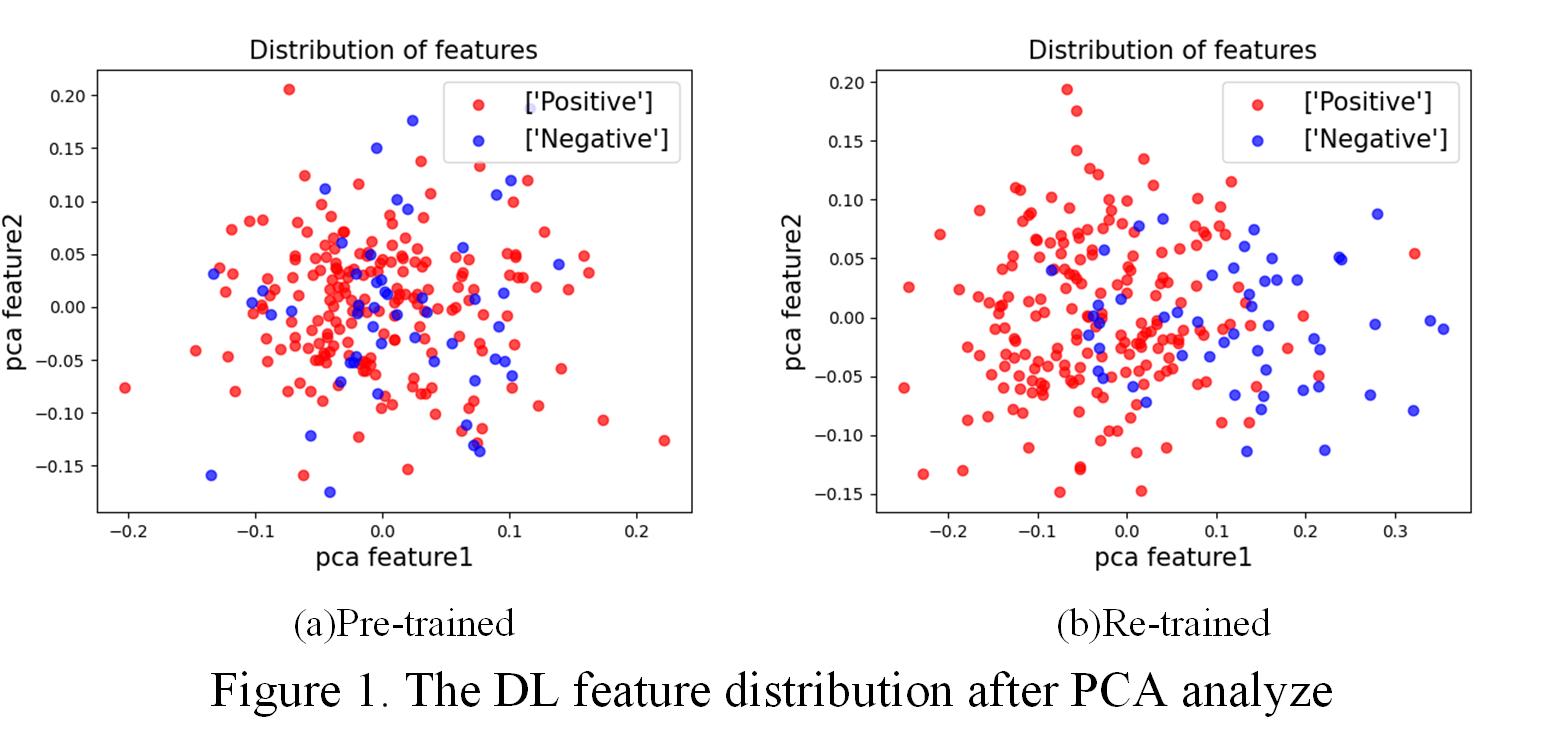
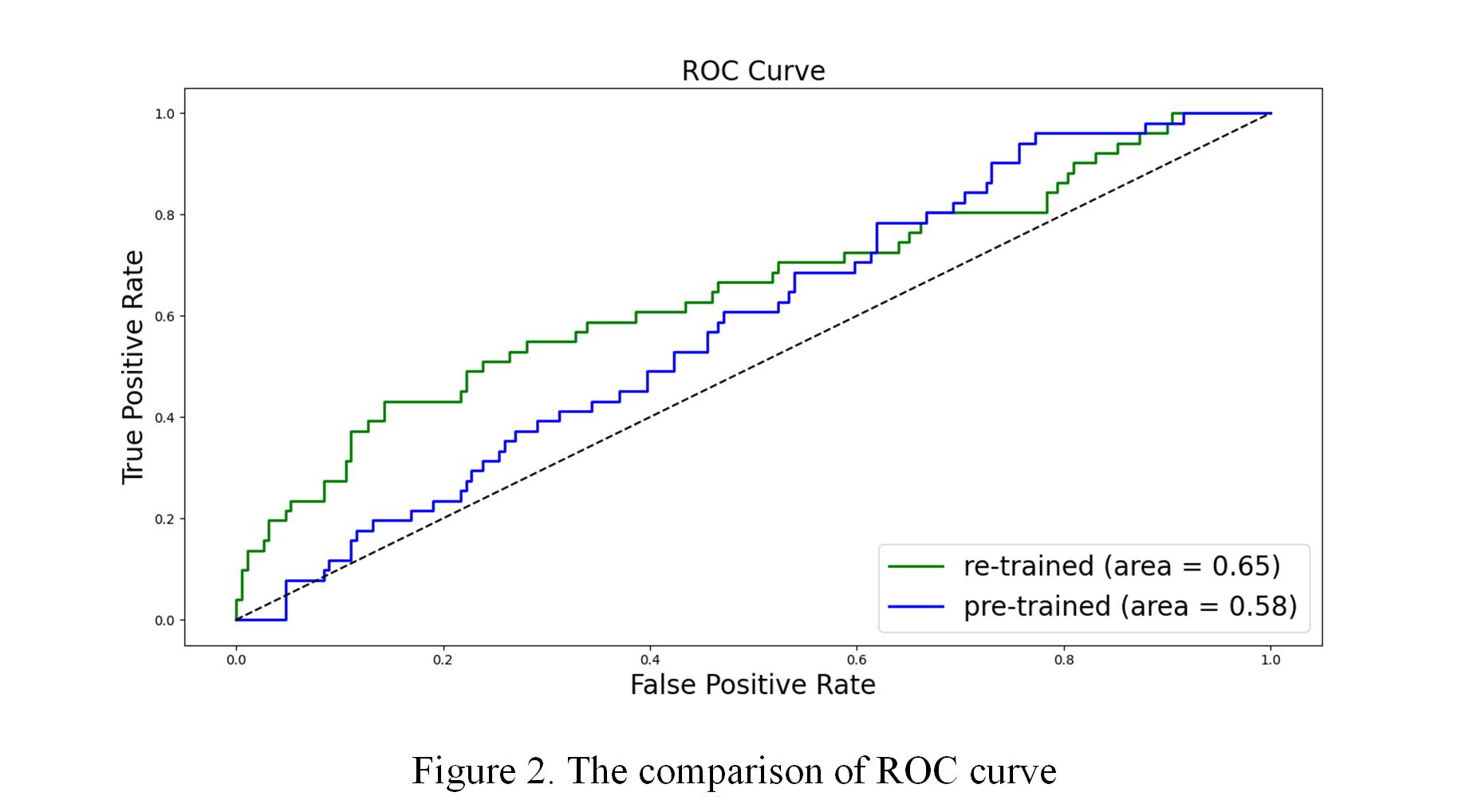
Conclusion
Both the pre-trained model and re-trained model have the potential to predict radiation pneumonitis, however, a re-trained model is preferable.