6
patients with FIGO stage IB2 and IIB cervical cancer had 3 MR scans each, with at least a week between scans. The scans were
acquired on a single MR machine. At each scan, a T2-weighted image was taken at the start and end of
the exam and Dixon sequences were sequentially
acquired over 10 minutes. For this study, T2W
images at the start and end of the scan and the first and last Dixon sequences
in the scan were included, resulting in 4 images per scan. This selection
results in a total of 36 test-retest pairs including both T2W and Dixon sequences.
The GTV was contoured by a single observer
on the start and end T2W images, and transferred through rigid registration to
the start and end Dixon sequence. For each image, radiomic
features were extracted from 2 regions: the GTV and a peritumoral region which extends from the GTV surface to 1.8mm (using the
start GTV as reference). The regions are shown in Figure 1.
PyRadiomics
was used to extract first-order, shape, and texture radiomic features from the
original T2W and Dixon images, and their Laplacian of Gaussian-filtered (with σ
= 3, 4, and 5) and Wavelet-filtered counterparts. This resulted in 1127
features per image.
Spearman’s ⍴ was used to exclude features highly correlated with
volume (|⍴| ≥ 0.9). Of the remaining features, those with minimal
variation between the start and end of a single scan were selected as being
stable using Intraclass Correlation Coefficient, ICC (1,1) ≥ 0.9. Spearman’s ⍴ was then used to identify features that were not
correlated with each other, with features having |⍴| < 0.65 being considered acceptable.
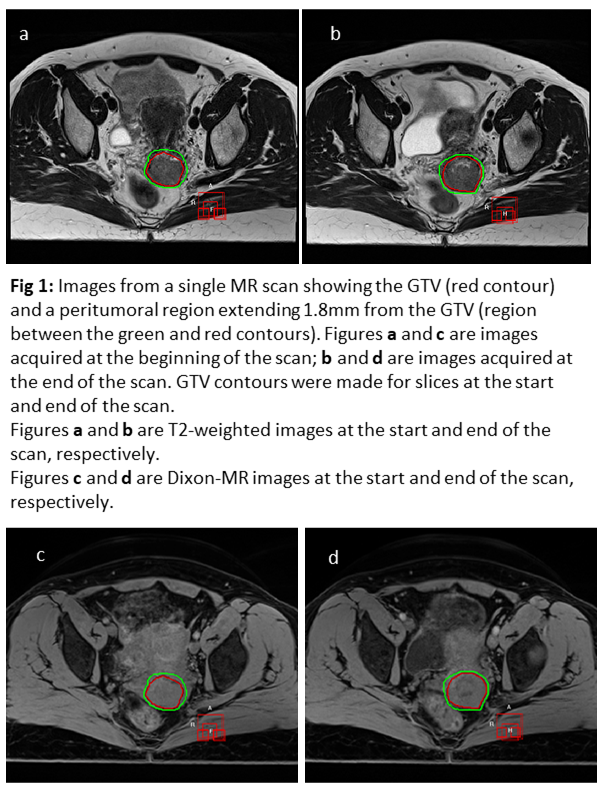