Associations of CT-based radiomics data with disease recurrence in early stage Lung cancer patients
Alexandra Giraldo Marin,
Spain
PO-1775
Abstract
Associations of CT-based radiomics data with disease recurrence in early stage Lung cancer patients
Authors: Alexandra Giraldo Marin1, Marta Ligero2, Alejandro Seoane3, Monica Ramos1, Marc Simo4, Jordi GIralt5, Manuel Escobar6, Perez-Lopez Raquel7
1Vall D´Hebron Hospital, Radiation Oncology, Barcelona, Spain; 2Vall D’Hebron Institute of Oncology, Radiomics Grroup, Barcelona, Spain; 3Vall D´Hebron Hospital, Medical Physics, Barcelona, Spain; 4Vall D´Hebron Hospital, Nuclear Medicine, Barcelona, Spain; 5Vall D´Hebron, Radiation Oncology, Barcelona, Spain; 6Vall D´Hebron, Radiology, Barcelona, Spain; 7Vall D’Hebron Institute of Oncology, Radiomics Group, Barcelona, Spain
Show Affiliations
Hide Affiliations
Purpose or Objective
To develop an
association between CT-based radiomics score and clinical prognostic factors
capable of predicting recurrence free survival (RFS) in patients with lung
lesions <5cm
Material and Methods
We analysed
data from 62 patients and 66 lesions treated with SABR from January 2015 to February 2019 in
our institution. 4D
PET-CT images were used for treatment planning, however, only CT images were
used for extracting Radiomics
features. The dose and number of fractions were selected according to our institution protocol.
Retrospectively, a RFS predictive signature was
derived. LASSO-Cox regression was implemented for feature selection and
univariate and multivariate Cox-proportional Hazard regression were implemented
with radiomics features and prognostic clinical factors. Kaplan-Meier (KM)
curves were implemented to evaluate the prognostic value of the signature. A
nomogram was developed for the clinical radiomics model.
Results
Patient’s characteristics are displayed in Table 1. The LASSO-Cox selected three radiomics features as
relevant for RFS prediction from GLCM and GLSZM matrices. The radiomics model
associated with RFS with a Concordance index (CI) of 0.63[0.55- 0.72]. Four
clinical factors were selected by LASSO (body mass index, Karnofsky status,
chronic obstructive pulmonary disease, and previous thorax irradiation). The
clinical model showed a similar predictive capacity as radiomics only (CI =
0.68[0.57- 0.78]). The integration of radiomics with clinical data improved the
predictive value to a CI=0.84[0.78-0.9]. KM curves showed significant
differences in RFS betwe
en low, medium and high clinical radiomics score
(Figure 1).
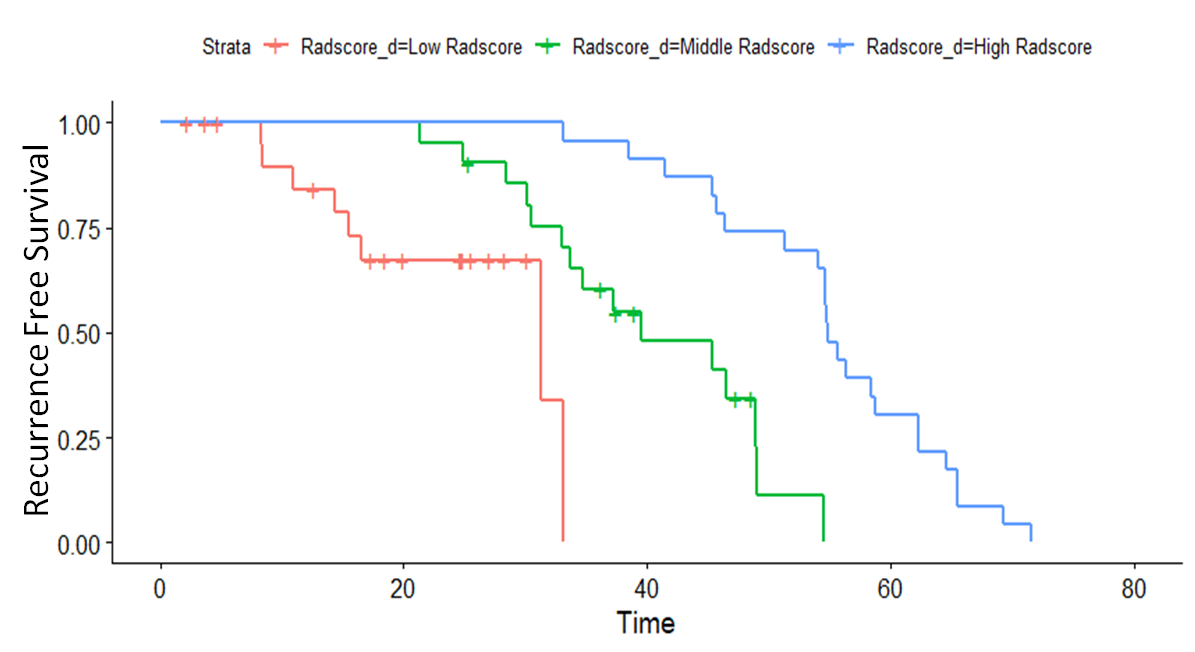
Conclusion
This study suggest that CT-based radiomics score
associated with prognostic clinical
factors might be a useful tool to improve the prediction of outcomes in
patients with lung lesions treated with radical intention. Validation of the
score is needed.