Independent validation of a PET radiomic model predicting outcome after Radiotherapy for HN cancer
PO-1760
Abstract
Independent validation of a PET radiomic model predicting outcome after Radiotherapy for HN cancer
Authors: Martina Mori1, Chiara Deantoni2, Michela Olivieri1, Emiliano Spezi3, Anna Chiara2, Simone Baroni2, Maria Picchio4, Antonella Del Vecchio1, Nadia Di Muzio2, Claudio Fiorino1, Italo Dell'Oca2
1IRCCS San Raffaele Scientific Institute, Medical Physics, Milan, Italy; 2IRCCS San Raffaele Scientific Institute, Radiotherapy, Milan, Italy; 3Velindre Cancer Centre, Medical Physics, Cardiff, United Kingdom; 4IRCCS San Raffaele Scientific Institute, Nuclear Medicine, Milan, Italy
Show Affiliations
Hide Affiliations
Purpose or Objective
To
externally validate 18Fluorodeoxyglucose Positron Emission
Tomography (18F-FDG-PET ) radiomic scores recently suggested by
Martens et al. (EJNMI Research (2020) 10:102) for the prediction of local recurrence
(LRFS), distant metastasis (DRFS) and overall survival (OS) in patients with oropharyngeal
cancer treated with chemo-radiotherapy.
Material and Methods
Outcome
data and pre-radiotherapy PET images of 78 patients (G1/G2=3; G3=13; G4=62) treated
at our Institute between 2006 and 2020 according to an internal protocol
delivering moderate hypo-fractionation (66 Gy in 30 fr) were available. Image
processing (voxelization and binning) was performed according to the procedures
described by Martens et al. and tumors were segmented using a previously
validated semi-automatic method with the software MIM (v.6.9.6). Four-hundred
and fifty radiomic features (RF) were extracted according to IBSI (Image
Biomarker Standardization Initiative) guidelines, using the SPAARC software (Whybra et al. Sci Rep 9:9649(2019)). Martens
et al. condensed the predictive RF in 8 independent meta-factors (F), consisting
of a combination of selected RF with variable importance weight. In particular,
two F named F1 (combining least-axis-length, non-uniformity,
high-dependence-of-high-grey-levels) and F5 (asphericity, major-axis-length,
inverse-compactness and inversed-flatness) were suggested as highly predictive
of OS. Then, F1 and F5 were tested in predicting OS on our population by Cox
Regression. An index combining both F was also computed and used to stratify
risk according to the best cut-off derived from the ROC analysis. Kaplan Meier
test was finally performed.
Results
Among
78 patients, 3, 11 and 22 events for LRFS, DRFS and OS were registered in our external
population. In Martens et al, RF selected as predictive were found only for
LRFS and OS prediction. Due to the small number of LRFS events in our
population, only OS prediction F may be tested. F1 and F5 derived from our
population were confirmed to be associated to OS (p=0.028 and 0.080
respectively). The prognostic index derived from Multivariate Cox analysis
combining F1 and F5 was tested with ROC analysis, showing AUC=0.68 (95%CL=0.56-0.78)
and p=0.006. According to the Youden criterion the best cut-off for the
combined index was >0.48, showing a high ability in patients stratification,
as depicted by the Kaplan Meier curve reported in the Figure below (p=0.001, HR=4.1,
95%CI=1.78-9.54). The 5-year OS were 91% (95%CI: 82-100%) vs 63% (48-80%) for low and high-risk groups respectively.
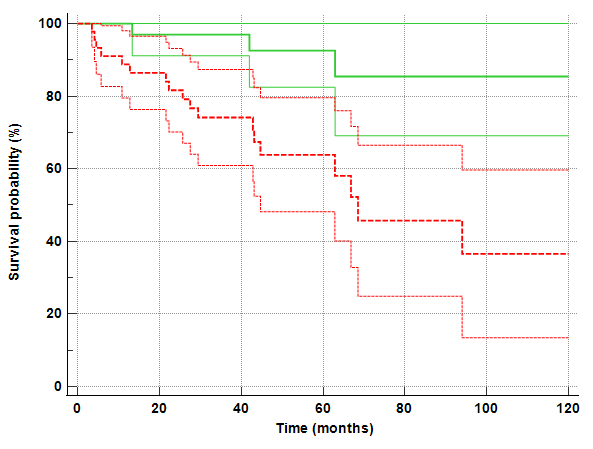
Conclusion
Previously
published radiomic scores were independently confirmed in predicting OS,
despite different Institute/scanners,
delivered dose and patient’s characteristics. Multiple validations like the
current one may help in corroborating the generalizability of radiomic-based
models, allowing for personalized risk stratification and optimization of personalized
cancer care.