Performance assessment of radiogenomics machine learning models for stratifying prostate cancer risk
Nerea Payan,
United Kingdom
PO-1758
Abstract
Performance assessment of radiogenomics machine learning models for stratifying prostate cancer risk
Authors: Neree Payan1, Ross G. Murphy1, Suneil Jain2,1, Alan R. Hounsell3,1, Sarah Osman1,3, Joe M. O'Sullivan2,1, Kevin M. Prise1, Conor K. McGarry3,1
1Queen's University, Patrick G. Johnston Centre for Cancer Research , Belfast, United Kingdom; 2Northern Ireland Cancer Centre, Belfast Health and Social Care Trust, Department of Clinical Oncology, Belfast, United Kingdom; 3Northern Ireland Cancer Centre, Belfast Health and Social Care Trust, Department of Radiotherapy Physics, Belfast, United Kingdom
Show Affiliations
Hide Affiliations
Purpose or Objective
We have previously established the potential of planning CT-based (pCT) radiomic models for prostate cancer (PCa) risk stratification [1]. In this study, we investigated the additional value of combining pCT-based radiomic with genomic data for PCa risk group (RG) classification and Gleason Score (GS).
Material and Methods
We included 184 patients with prostate cancer in this study. We extracted 5983 radiomics features from pCT images, and combined them with 19453 gene expression features from microarray. Following our previously published methodology [1], GS and RG classifications were performed using logistic regression. Models were created using radiomic (R) and genomic (G) features individually as well as with a separate combined (R+G). Algorithms were trained on two thirds of the dataset (124 patients) and tested on the remaining third (60 patients). The model dimensions were reduced by removing the highly correlated variables (with Pearson correlation r > 0.8) and by performing feature selection using Least Absolute Shrinkage and Selection Operator (LASSO) and ElasticNet regularisations. Models were optimised using 10 folds stratified cross-validation, replicated 100 times within the training set. Area Under the ROC Curves (AUC) were used for model optimisation and accuracy, Youden Index (YI) and F1-scores were calculated. Groups of GS6, GS7(3+4), GS7(4+3) and GS8 to 10 were considered. Thirty-two patients had tumours with GS6, 41 patients had GS7(3+4), 32 patients had GS7(4+3) and 79 patients had GS8 or higher. Prostate tumours were also classified as low, intermediate or high risks based on GS, initial PSA and TNM stage. Four patients had low risk cancer, 36 had intermediate risk and 144 had high risk cancer.
Results
Table 1 summarises the AUCs, accuracy, YI and F1-scores for the training and test results, and the number of selected features in each model. Classification between GS6 vs >GS6 (N= 32 vs 152) showed AUCR = 0.55, AUCG = 0.63 and AUCR+G = 0.63 within the test set. Classification between GS7(3+4) vs GS7(4+3) (N=41 vs 32) showed AUCR = 0.54, AUCG = 0.69 and AUCR+G= 0.68 on the test set. Finally, AUCR = 0.57, AUCG = 0.62 and AUCR+G = 0.65 were observed on the test set for the classification of GS7 vs >GS7 (N=73 vs 79). Regarding the risk group classification, only classification between intermediate and high risk (N=36 vs 144) was performed due to the reduced number of low risk patients in this cohort. For these subgroups, the test results showed AUCR = 0.63, AUCG = 0.79 and AUCR+G = 0.79.
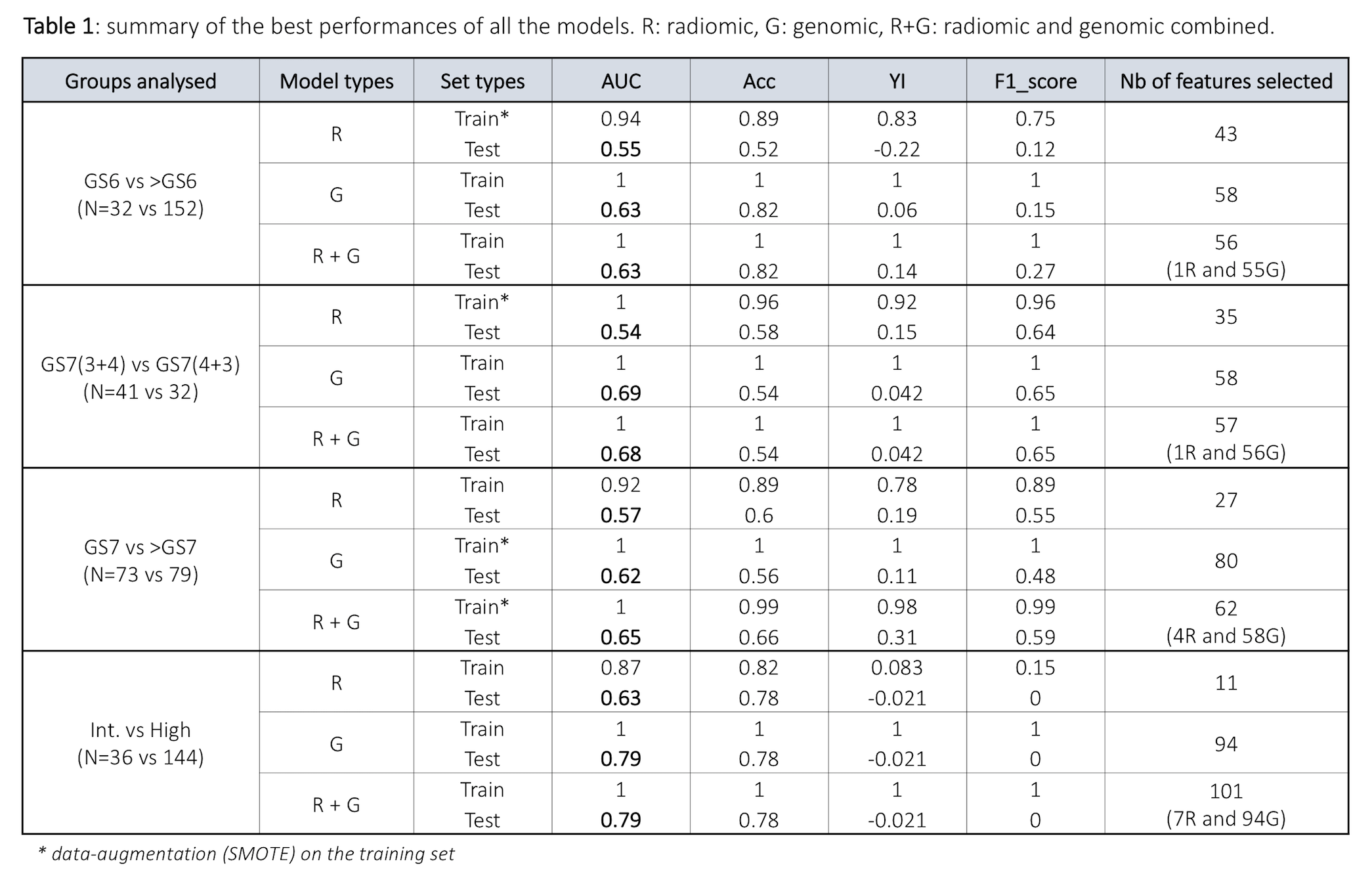
Conclusion
Genomics models outperformed the radiomics models for risk stratification in prostate cancer. Our combined radiogenomics model improved performance for the classification between GS7 and GS higher than 7 (>GS7). External validations are warranted to verify these findings.
[1] Osman et al. Int. J. Radiat. Oncol. Biol. Phys. 2019; 105(2):448-456