Evaluation of three AI-based CT auto-contouring systems for head&neck, thorax and pelvis
PD-0315
Abstract
Evaluation of three AI-based CT auto-contouring systems for head&neck, thorax and pelvis
Authors: Marta Casati1, Mauro Loi2, Chiara Arilli1, Livia Marrazzo1, Cinzia Talamonti1,3, Margherita Zani1, Antonella Compagnucci1, Gabriele Simontacchi4, Vanessa Di Cataldo5, Isacco Desideri3, Pierluigi Bonomo4, Nicola Franza6, Davide Raspanti7, Roberto Pellegrini8, Lorenzo Livi3,2, Stefania Pallotta3,1
1Azienda Ospedaliero Universitaria Careggi, Medical Physics, Florence, Italy; 2Azienda Ospedaliero Universitaria Careggi, Radiotherapy Unit, Florence, Italy; 3University of Florence, Department of Experimental and Clinical Biomedical Sciences, Florence, Italy; 4Azienda Ospedaliero Universitaria Careggi, Radiation Oncology, Florence, Italy; 5Florentine Institute of Care and Assistance (IFCA), Radiation Oncology, Florence, Italy; 6DosimETrICA, DosimETrICA, Nocera Inferiore (SA), Italy; 7Temasinergie S.p.A., Radiotherapy and Diagnostic Radiology, Faenza (RA), Italy; 8Elekta AB, Global Clinical Science, Stockholm, Sweden
Show Affiliations
Hide Affiliations
Purpose or Objective
To evaluate both performances and clinical acceptability
of auto-contours generated by three AI-based software on 18 CT studies: 6 Head
and Neck (H&N), 6 Thorax (T), and 6 Pelvis (P).
Material and Methods
The structures listed in table 1 have been assessed,
for each test study. The evaluated AI-contours were generated with deep
learning algorithms by: Contour Protégé AI (Protégé) v. 2.0 (MIM software Inc.
7.1.5), Limbus Contour (Limbus) v. 1.3.0 (Limbus AI Inc.), and Admire v. 3.28 (prototype
by Elekta) software. The type and number of contours automatically contoured by
the three software are different. Lymph nodes were not evaluated. For the Pelvis,
we also compared the performances of AI-based with atlas-based segmentation
approaches. For this purpose two MIM atlases: a proprietary atlas (High-Risk
Prostate, HRP) and an in-house developed atlas (AOUC) [1] were employed,
invoking them from an in-house, multi-subject customized workflow, in which
registration parameters and post-processing options were optimized.
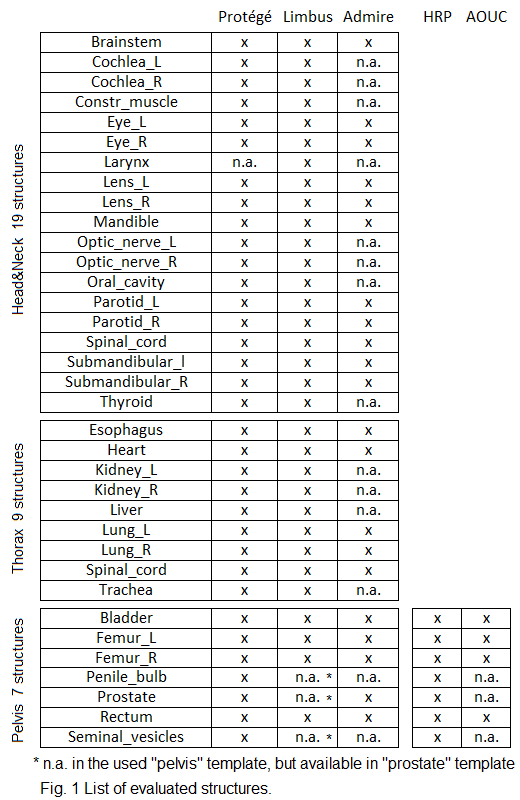
Each contour (including manual) was visually evaluated
in a blinded test by a Radiation Oncologist (RO) (other than the reference one),
assigning a score proportional to the degree of corrections needed for clinical
suitability: 0 (contour acceptable without editing); 1 (minor revision
required); 2 (further revision needed).
Results
For each district, the percentage of evaluated
structures scored 0, 1 or 2 are reported in figure 2a.
The percentage of evaluated structures for which no
corrections or minor corrections were needed are reported in fig. 2b.
For all districts, more than 79% of DL contours are
acceptable or need minor corrections (Fig. 2b).
DL contours generally have a higher degree of clinical
acceptability than atlas-based contours. Although certain atlas-based contours
sometimes require even major revision, we improved MIM HRP atlas contours
quality by optimizing the workflow image-registration options and
post-processing steps. Results further improved by using AOUC atlas, created and
optimized in-house: the fraction of contours scored 0 or 1 reaches 92%,
comparable to DL-generated contours.
Conclusion
DL-based algorithms represent a turning point in the
field of auto-contouring and produce high quality contours. Even if some
corrections are needed before clinical use, in clinical practice, important
time-savings may be obtained, if no or minor corrections are needed.
To date, evaluated deep-learning algorithms are
capable to produce high quality contours, in most cases clinically acceptable
or prone to be quickly edited with minor revision.
[1] Casati et Al. DOI: 10.1002/acm2.13093