A comparison of three commercial AI contouring solutions
Konstantinos Ferentinos,
Cyprus
PO-1491
Abstract
A comparison of three commercial AI contouring solutions
Authors: Paul Doolan1, Stefanie Charalambous2, Yiannis Roussakis1, Agnes Leczynski2, Konstantinos Ferentinos2, Iosif Strouthos2, Efstratios Karagiannis2
1German Oncology Center, Department of Medical Physics, Limassol, Cyprus; 2German Oncology Center, Department of Radiation Oncology, Limassol, Cyprus
Show Affiliations
Hide Affiliations
Purpose or Objective
To compare the performance of three
commercial artificial intelligence (AI) contouring solutions. To determine if
they offer an advantage compared to manually-drawn expert contours, both in
terms of quality and time, and to observe if use of such contours translates to
an impact on the dosimetry of the plan.
Material and Methods
In this work three commercial AI contouring solutions were tested:
DLCExpert from Mirada Medical (Mir) (Oxford, UK); MVision (MVis) (Helsinki,
Finland); and Annotate from Therapanacea (Ther) (Paris, France). A total of 80
patients were assessed: 20 from each of the four anatomical sites (breast, head
and neck, lung, and prostate). The AI-generated contours were compared to
expert contours drawn by Radiation Oncologists following the RTOG and MacDonald et
al (2016) protocols. Differences in
the structures drawn by the commercial systems were identified. The times to
generate the expert contours, as well as the time to correct the AI-generated
contours, were recorded. Each AI contour was quantitatively compared to its
corresponding manually-drawn expert contour using the DICE index, concordance
index and Hausdorff distance. The AI contours from each software were overlaid
on the planned radiation dose to observe if the different contours result in
any dosimetric differences.
Results
For the expert contours, the mean
time required to manually draw the twenty cases for each site was: 22 mins
(breast); 112 mins (head and neck);
26 mins (lung); and 42 mins (prostate). The time taken to correct the AI-generated
structures was less than these durations and very similar among the three
commercial solutions, for all sites. The number of structures contoured by the manually-drawn
Expert and Mir/MVis/Ther solutions was: 10 and 9/17/30 for breast; 33 and 29/46/46
for head and neck; 7 and 5/13/8 for lung; 12 and 7/16/18 for prostate. Compared
to the structures that are routinely contoured in our clinic, there were a
number of structures missing from each model. Conversely, in the prostate model
MVis contours the lymph nodes, while Ther contours the lymph nodes for breast,
head and neck and lung models (none of which are not routinely contoured in our
clinic).
The DICE and
concordance indices were very high for all systems, across all sites. Figure 1 shows the DICE indices for breast, which had median DICE
values for Mir/MVis/Ther: Heart 0.91/0.96/0.92, Oesophagus -/0.79/0.82, Lung_L 0.98/0.96/0.97,
Lung_R 0.97/0.95/0.96, Breast_L 0.88/0.88/0.90, Breast_R 0.82/0.72/0.89, Liver 0.76/0.82/0.84,
SpinalCanal -/0.96/0.96. Figure 2 shows the corresponding Hausdorff distances.
Fig1: Breast DICE indices.
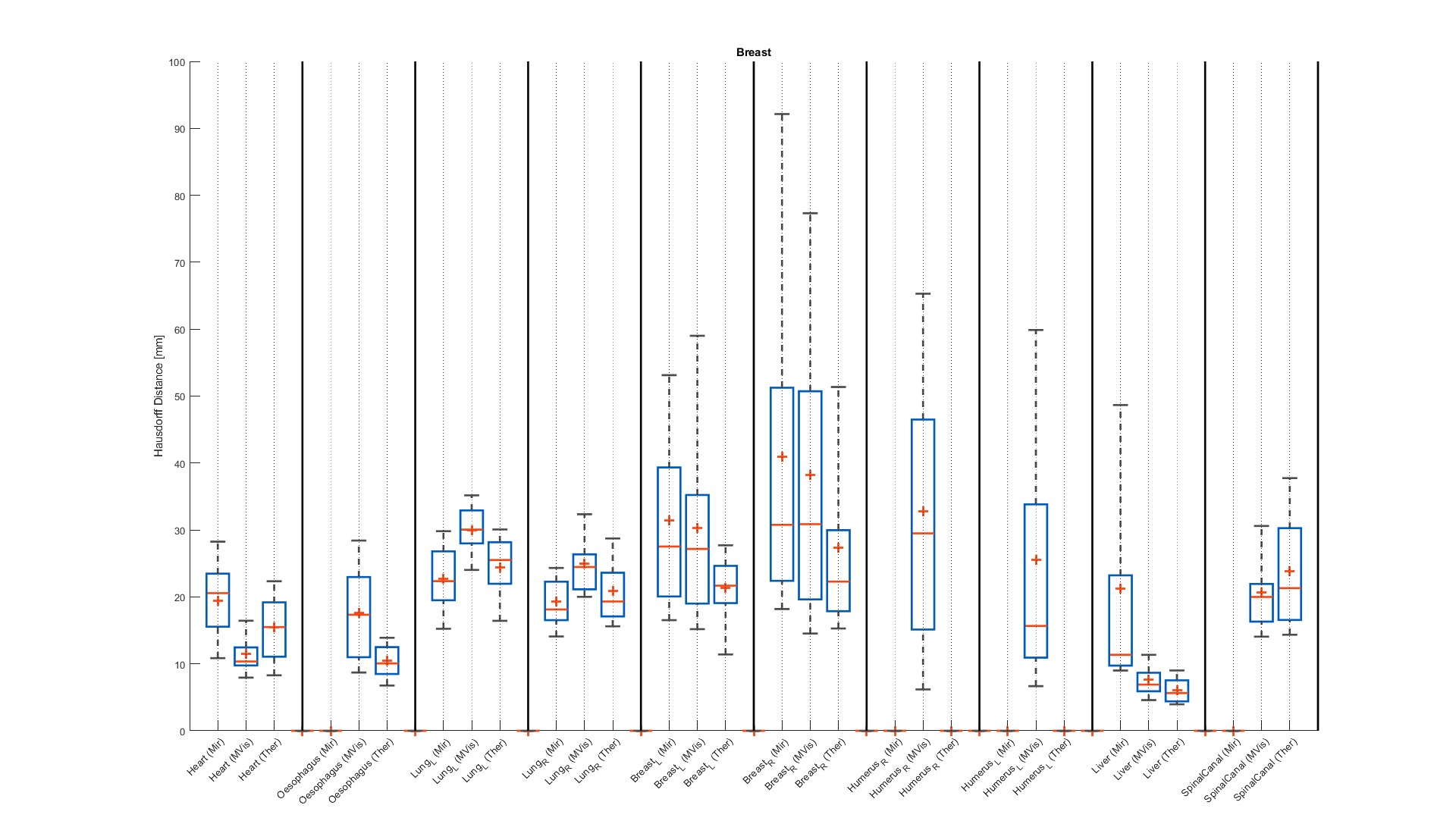
Fig2: Breast Hausdorff distances.
Conclusion
It can be concluded that all
three commercial AI contouring solutions provide excellent quality structures
across all anatomical sites. The AI-generated contours have consistently high
DICE indices and low Hausdorff distances. The time to correct the AI contours
was less than contouring the structures manually, for all systems.