Time-dependent machine learning survival prediction model of brain metastases with MRI radiomics
Simon Joseph Clément Crête,
Canada
PD-0735
Abstract
Time-dependent machine learning survival prediction model of brain metastases with MRI radiomics
Authors: Simon Joseph Clément Crête1, Nicolas Bergeron Campbell1, Ricky Hu2, Jacob Peoples3, Michael Yan4, Tim Olding4, Kathrin Tyryshkin5,3, Amber L. Simpson3,6, Fabio Ynoe de Moraes4
1Queen's University, Computer Engineering, Kingston, Canada; 2Queen's University, School of Mediciine, Kingston, Canada; 3Queen's University, School of Computing, Kingston, Canada; 4Queen's University, School of Medicine Department of Oncology, Kingston, Canada; 5Queen's University, School of Medicine Department of Pathology and Molecular Medicine, Kingston, Canada; 6Queen's University, Department of Biomedical and Molecular Sciences, Kingston, Canada
Show Affiliations
Hide Affiliations
Purpose or Objective
Brain metastases diagnosis severely impacts the prognosis of
cancer patients. Accurate prediction of survival time would enhance prognosis
and treatment selection. The use of machine learning utilizing magnetic
resonance imaging (MRI) radiomic features has been investigated in patients
with brain metastases, but these studies do not undertake time-dependent
analysis. We propose a time-dependent model using radiomic features extracted
from MRI that has the potential to deliver higher accuracy prognostication in
this population.
Material and Methods
Patients
diagnosed with brain metastases treated at our institution from 2016-2020 were
included in the study. Clinicopathological variables were collected and
analyzed for association with survival. Treatment MRI were pulled from the
health information system, segmented by the clinical team, and analyzed using
quantitative techniques. Image intensities were normalized based on z-score,
and voxel spacing was resampled to 0.9x0.9x0.9mm. Standard radiomic features
(116) were extracted using PyRadiomics. These features were grouped by their
high collinearities with other features using their variance inflation factor. Features
with the highest variance were selected from each group. The Random Survival
Forest model from the open-source PySurvival library was trained using
radiomic features and significant clinical variables to predict overall survival.
Before training, 10% of the data was split as a holdout testing set. The model
used four-fold cross validation (CV) on the remaining data to prevent
overfitting, with a 75% training and 25% validation split within each fold. Optimal
hyperparameters for this model were established using grid search, and those
yielding the highest concordance index (C-index) were selected. The metrics
used to evaluate the final model were average C-index and integrated Brier
score (IBS).
Results
In total, 157 patients diagnosed with brain metastases
(mixed histologies) were analyzed. Sex (p = 0.018) and 14 radiomic features were
included in the final model. The model
outputs a survival curve, hazard curve, and risk score. The survival model
obtained a C-index of 0.74 and IBS of 0.17 and C-index of 0.675 and IBS of 0.175,
on the training and validation data, respectively. The holdout test set
obtained a C-index of 0.674 and IBS of 0.168. Figure 1 displays validation
metrics, a) shows the Brier Score for every time unit and the 0.25 threshold,
b) shows the predicted survival vs. the actual survival with the confidence
interval of the prediction.
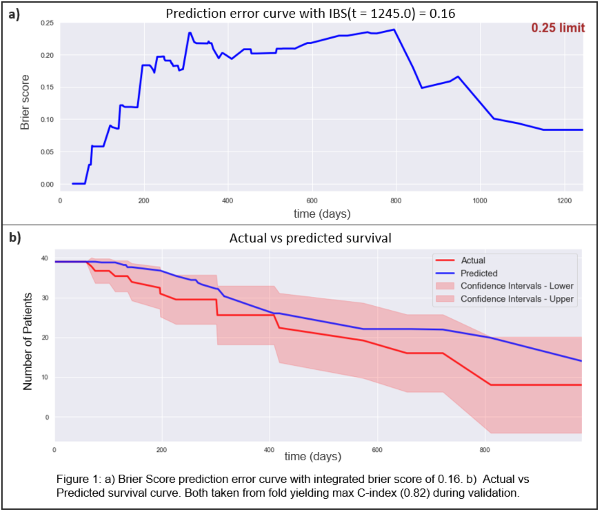
Conclusion
The proposed method utilized radiomic features to train a model
for brain metastases survival estimation. Results showed that the model produces
accurate and meaningful survival predictions. Our findings suggest that brain
metastases radiomic features might be applicable for predicting survival in
patients with brain metastases.