ESTRO 2023 RTT Track
Trends in dosimetry planning - Mini Oral Session
ESTRO 2023 demonstrated once again the great importance of software development in radiotherapy along with the growing role and advanced practice required of radiation therapists (RTTs). The role of the RTT has grown extensively due to the increasing number of advanced techniques that they must use and the complexity of these techniques, which require RTTs to become principal actors rather than mere performers (according to the idea of professional autonomy). Therefore, an increasing range of skills (both professional and human), competencies (augmented by additional competencies and new skills) and knowledge are needed.
Multidisciplinary professional experiences are supported by new treatment planning systems and other complex software, the use of which increases the quality of treatment by reducing the time required for it. This consequently enables greater accuracy and reduced toxicity in the provision of the best treatment to patients. Recently, advanced treatment modalities, such as intensity-modulated radiotherapy and volumetric arc therapy (VMAT), have been applied to deliver higher doses to tumour areas while reducing the therapeutic doses to normal organs compared with conventional 3D-conformal radiotherapy. However, the ability to produce an optimal plan with the application of these advanced treatment methods varies with the experience of the planner, and a time-consuming planning task must be repeated until the treatment goal is achieved. Therefore, studies have been conducted to improve the efficiency and quality of treatment planning while reducing planning time and effort by using knowledge-based techniques for dose prediction in radiotherapy.
Automation has been shown to provide great benefits: an automated planning system that uses dose prediction with deep learning and dose mimetic optimisation has been implemented to enable efficient investigation and optimisation (DLO) of treatment parameters while sparing organs-at-risk (OARs) and nearby healthy tissues. Through collaboration between the Arnhem Institute of Radiotherapy (The Netherlands) and Ray Search Laboratories (Stockholm, Sweden), DLO has been implemented as a decision support tool for dosimetrists who need to optimise treatment plans further without compromising target coverage (especially in cases of head and neck cancer).
As radiation treatment technology advances, the treatment outcomes for cancer patients are gradually improving. Deep-learning methods have proven effective in various fields, such as automatic segmentation, image registration, and predictions of respiratory motion and toxicity. Radiotherapy treatment planning based on the predicted dose according to deep learning will improve the radiotherapy process by reducing the time required for planning while maintaining or increasing the quality of the plan.
Therefore, new radiotherapy quality assurance programmes on normal tissue dose-volume constraints should be updated to ensure safe and consistent application. This means, for example, that the planning parameters for stereotactic body radiation therapy accreditation for oligometastatic disease must be reconsidered with the evaluation of an updated and more conservative consensus on normal-tissue dose-volume constraints, as the National Radiotherapy Trials Quality Assurance Group (Northwood, UK) has done, although this type of comparison should not demonstrate a significant impact on planning. Or, further, we can compare the dosimetry of a conventional linear accelerator (Linac) with that of an integrated MR-Linac regarding accelerated partial breast irradiation (APBI). The experience of staff at the Olivia Newton-John Cancer, Wellness and Research Centre (Heidelberg, Australia) indicates that clinically acceptable APBI plans generated for an MR-Linac are comparable with conventional VMAT plans.
To minimise the involvement of normal tissues, considerable efforts have been made to develop daily position verification techniques.
The current radiation therapy paradigm requires that treatment be planned based on a set of snapshot data of the patient's anatomy taken at the time of simulation. During the course of treatment, this snapshot may vary from the initial simulation. Although there is the ability to view images of patients inside the treatment room with technologies such as cone-beam computed tomography, the current state-of-the-art is largely limited to rigid body matching and does not account for any geometric deformations of the patient's anatomy. A plan that was once in tune with the initial simulation may become suboptimal as treatment progresses, unless better technologies are applied. Adaptive radiotherapy (ART) is an evolving paradigm that seeks to fill this gap by taking into account ongoing changes in the patient's anatomy and/or physiology during treatment, enabling increasingly precise targeting of the disease. ART relies on several components working in concert, namely in-room treatment image guidance, deformable image registration, automatic retouching, plan evaluation and re-optimisation, dose calculation, and quality assurance. Several studies have explored how an ART solution could improve the current state-of-the-art in radiation therapy; some centres have even implemented online adaptation clinically.
Online adaptive radiotherapy (oART) has been introduced into clinical practice, but its use has been limited due to the need to reschedule daily verification images because of variation in anatomical information. Effective rescheduling strategies are urgently needed: several studies suggest that the plan-library (PL) approach should be used to handle daily anatomical variations. The Policlinico Universitario A. Gemelli IRCCS Radiotherapy Foundation (Rome, Italy) has demonstrated how the PL-based hybrid approach that is obtained with oART plans can be implemented in the clinical practice of cervical cancer MRI. This approach could offer a solution to reduce the burdensome daily workflow of oART while maintaining the use of a reduced margin in the planning target volume, paving the way for more efficient dose escalation and OAR savings.
Limitations in the development of routine oART are due to time-consuming manual segmentation and variation in accuracy among observers. Time trends for oART on an MR-Linac 1.5 are available from the study conducted by the University Medical Centre Utrecht for prostate cancer, rectal cancer, or lower motor neuron disease (LMN) by RTTs trained from 2020. The duration of the preparation phase (DPP) was defined as the interval between the start of the pretreatment scan and the start of the position verification scan, representing the duration of the first MR scan, image registration, matching, and manual fitting.
This study showed that the DPP decreased over time for the treatment of prostate and rectal cancer and remained stable for the treatment of LMN. The introduction of RTT-driven workflow did not increase the DPP.
This new implementation provided an opportunity to evaluate the roles of each member of the multidisciplinary team (RTTs, medical physicists and radiation oncologists (ROs) in order to optimise the use of expertise to maximise efficiency and to develop new training strategies for the transition from RO-guided to RTT-guided contouring, particularly on a hybrid platform such as an MR Linac, which is heralded as a milestone in radiotherapy. A great deal of work has been done by the radiotherapy team at the Princess Margaret Cancer Centre (Toronto, Canada), where a practice-based training strategy was developed for RTT-guided MR-Linac prostate ART to enable RTT-guided workflow for MR-Linac treatment. This model can be adapted to other anatomical sites to maximise the efficiency of MR-Linac radiotherapy. In phase one, RTTs independently contoured the target and OARs on T2-weighted MR images of 11 prostate patients who had been previously treated with an MR-Linac. The RO reviewed qualitatively the RTT-generated contours through the use of a five-point Likert scale; a score of 4 or 5 was considered positive, and a 90% pass rate was required to complete phase one. Phase two involved contouring, plan generation, and online assessment by the RTT under the supervision of the RO during the delivery of adaptive treatment fractions; to advance, participants had to achieve an autonomy score (no direction required by the RO) on 10 cases (minimum eight patients). The final phase of training involved operator-guided autonomous contouring and planning by the RTT, supported by offline operator review of the contouring and plan before administration of the next fraction.
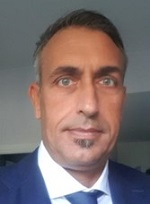
Paolo BROSSA
RTT coordinator, University of Turin Radiotherapy
RTT coordinator, S. Giovanni Antica Sede Radiotherapy
A.O.U. Città della Salute e della Scienza Hospital, Turin, Italy