Editors’ pick
Use of knowledge-based DVH predictions to enhance automated re-planning strategies in head and neck adaptive radiotherapy - PDF Version
Elisabetta Cagni, Andrea Botti, Agnese Chendi, Mauro Iori and Emiliano Spezi
Phys Med Biol 2021 Jun 23;66(13). doi: 10.1088/1361-6560/ac08b0.
What was your motivation for initiating this study?
As part of my PhD project that I am undertaking in the School of Engineering of Cardiff University (UK), I am investigating several aspects of automation in radiotherapy treatment plans. This paper describes part of the project, and it explains work that was developed in the Medical Physics Unit of the local health authority’s Research Institute in Advanced Technologies and Healthcare Models in Oncology (AUSL-IRCCS) of Reggio Emilia (Italy). This is the hospital where I work as a medical physicist. In the institute we have experience with knowledge-based tools for treatment plans (KBP) and we decided to investigate one aspect of these: the application of KBP to adaptive radiotherapy (ART).
This paper presents a novel application of a commercial KBP tool for radiotherapy planning, which estimates the sparing of potential organs at risk (OARs) in the replanning strategy for head and neck ART.
External beam radiotherapy is gradually evolving towards real-time ART, which is becoming a new paradigm in radiation oncology. ART has the clinical rationale that its use enables plan adaptation that can reduce toxicity to normal tissue and OARs and/or improve tumour control. The frequency of re-planning in patients with head-and-neck cancer has been reported to vary from 32% to 70% depending on several criteria, such as patient weight loss, change in neck separation or poor fit of the immobilisation shell. However, at present, there are still several technical limitations in the application of ART as an automation and standardisation process. Some investigators are researching automated methods to predict the eventual need for re-planning, but more work is needed.
KBP tools can generate estimated dose‐volume histograms (DVHs) based on previous patient anatomy and dose distributions. Previously published studies have demonstrated the efficacy of KBP to create new plans for several cancer sites.
We investigated the feasibility of using KBP in a new application as part of the ART process to estimate the potential gain in OAR sparing during the treatment course for head-and-neck cases. If a significant relationship between KBP prediction and OAR sparing could be discovered, it could establish the need for plan adaptation based on OAR sparing and for automation of the process of re-planning itself.
What were the main challenges during the work?
Previously published studies have demonstrated that the efficacy of KBP is influenced by the quality of the data used for the training process, the regression applied to build the predictive models and the consistency that is found between new cases and the population used for the training. KBP training generally involves use of a database of manually generated treatment plans that can suffer from plan quality variation and inconsistency.
Use of the KBP tool produced two predicted DVH bounds with a 68% probability that the final DVH would fall between the two. To maximise the performance of KBPs (i.e. to reduce the difference between these two predicted DVH bounds), Pareto plan solutions, which were created with the use of a Trade-Off multicriteria Varian module, were used to train the KBP model. However, even after such training with Pareto optimal plans, a relevant KBP prediction uncertainty remained. The KBP tool could be considered a useful application in ART if KBP prediction uncertainties were required to be significantly smaller than, or at least comparable with, the predicted gain in OAR sparing.
What is the most important finding of your study?
The study demonstrated the feasibility of using the KBP tool to establish the need for plan adaptation based on OAR sparing to help to automate the process of re-planning. This study has shown that the prediction uncertainties when KBP tools trained with Pareto-optimal plans are used are sufficiently low for such tools to be used in ART. The paper presents a systematic workflow to identify effective OAR sparing in replanning strategies that are based on KBP prediction. We conclude that application of this KBP method to ART could give feasible estimations of OAR sparing.
What are the implications of this research?
The approach described in this work contributes to the automation of ART processes.
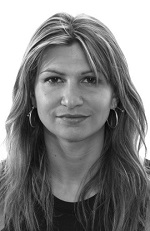
Elisabetta Cagni
Medical Physics Unit
Azienda USL-IRCCS di Reggio Emilia
Reggio Emilia, Italy.
&
School of Engineering
Cardiff University
Cardiff, United Kingdom.
elisabetta.cagni@ausl.re.it