PhD Research Report
Harnessing Magnetic Resonance Imaging for Real-Time Adaptive Radiotherapy - PDF Version
Abstract
The effect of radiotherapy is often diminished by changes in the patient’s anatomy during dose delivery. Tumour and organ movement, caused by respiratory, cardiac and gastrointestinal activity, result in underdosage of the tumour and unwanted exposure of healthy organs-at-risk. Through use of real-time adaptive radiotherapy, clinicians can aim to mitigate the impact of anatomical changes by adjusting the dose delivery in real-time. This can be achieved by gating the treatment beam, repositioning the patient using a robotic treatment couch or by dynamically adjusting the treatment beam using the linear accelerator’s multileaf collimator (MLC). All these techniques rely on the availability of up-to-date information about the patient’s anatomy. Recently, radiotherapy treatment machines with integrated magnetic resonance (MR) imaging have entered clinical practice. Use of these devices enables the continuous surveillance of the patient who is undergoing treatment without the need for implanted markers or additional imaging dose. My PhD project has explored the potential to harness the on-board MR imaging capabilities of the Elekta Unity 1.5 T MR-linear accelerator (linac) for real-time adaptive radiotherapy. In particular, it has investigated the feasibility, implementation and potential benefits of MLC tracking that is guided by 2D cine MR imaging.
Which institution were you affiliated with during your PhD?
I conducted my PhD thesis at the Institute of Cancer Research and The Royal Marsden National Health Service Foundation Trust in London.
What was the motivation for the topic of your PhD work?
The need to develop real-time adaptive radiotherapy techniques for the MR-linac was the main motivation for my PhD research. The prospect of guiding real-time adaptive radiotherapy with MR imaging was not only one of the driving forces behind the development of MR-guided treatment machines, but also a topic I personally found very interesting. I had investigated intrafractional motion management and MLC tracking for my Bachelor of Science and Master’s theses. As I was finishing my Master’s thesis in 2014, my supervisor Professor Uwe Oelfke hinted that our institute might be one of the first centres to install the Elekta Unity MR-linac. When he asked whether I was interested in researching MR-guided real-time adaptive radiotherapy as a PhD student, I quickly said yes.
What were the main findings of your PhD?
My PhD work can be split into three parts. At the beginning of my project, it was unclear whether it was possible to use MR-guided radiotherapy machines to deliver enhanced treatments to patients. Both the unique machine design and the presence of a strong magnetic field were thought to deteriorate the quality of treatments, in particular for anatomies that featured air-tissue interfaces. We conducted two planning studies to investigate the feasibility of creating lung radiotherapy treatment plans for the Elekta 1.5 T Unity MR-linac. These showed that accounting for the magnetic field during treatment plan optimisation enabled the design of clinically acceptable treatments (see Figure 1). Additionally, we found that the potential benefits of MLC tracking and treatment margin reduction were not inhibited by machine design or magnetic field.
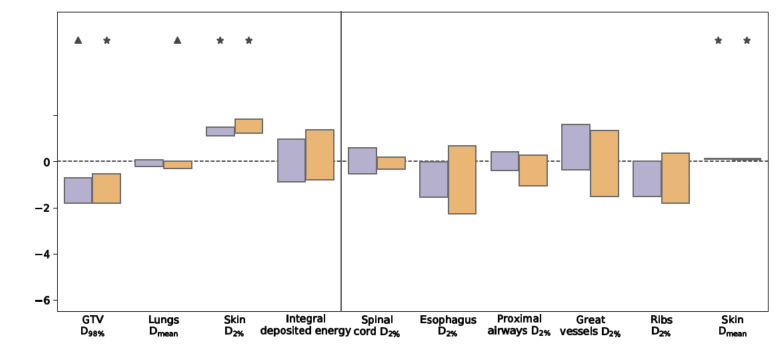
Figure 1: Box plots presenting the differences in the investigated dose-volume metrics and integral deposited energy due to the presence of a 1.5T magnetic field, either without tumour tracking (conventional: 1.5T - 0T) or with MLC tumour tracking (tracked: 1.5T - 0T). Each scenario was simulated for ten non-small-cell lung cancer patients. The magnetic field did not inhibit the ability to generate treatment plans that fulfilled the planning goals set by the Radiation Therapy Oncology Group 1021 guidelines. Furthermore, the ability to use real-time tumour tracking to decrease dose exposure of healthy tissue was not affected by the magnetic field. Figure adapted from Menten et al. (2016), Radiotherapy and Oncology 119(3), pp. 461-466.
In the second part of the project, we demonstrated that it was possible to localise automatically 3D volumes of interest in real time through use of 2D cine MR imaging. Through use of a template-matching algorithm that processed coarse 2D cine MR images, we were able to localise accurately kidneys and gallbladders in cancer patients and healthy volunteers and lung tumours (see Figure 2). We found that use of more complex algorithms or increasing the number and resolution of the 2D cine MR images that were used improved localisation accuracy only marginally.
.jpg.aspx;.pdf)
Figure 2: Comparison of four different techniques used to contour four different lung tumours automatically: multi-template matching (MTM), b-spline based deformable image registration (DIR), pulse-coupled neural network (PCNN) and scale-invariant feature transform (SIFT). The ground truth is marked in red, while the algorithmically derived contour is displayed in green. The accuracy of the simple template-matching algorithm is comparable with the inter-observer variability while it requires less than 1 ms to outline a lung tumour. Figure adapted from Fast et al. (2017), Radiotherapy and Oncology 125(3), pp. 485-491.
In the final part of my PhD project, we developed an online dose reconstruction tool that was able to calculate the dose that was actually delivered based on data that is routinely acquired during MR-guided radiotherapy. It employs 3D MR images, 2D cine MR images and treatment machine log files to calculate the delivered dose. It takes intrafractional motion and potential real-time treatment adaptation into account. We used the developed pipeline to measure anatomical changes and assess their dosimetric impact in 89 prostate radiotherapy fractions that were delivered with the MR-linac to our institute (see Figure 3). Thereby, we demonstrated that through this end-to-end workflow, we could reliably, non-invasively and automatically reconstruct the delivered radiotherapy dose.
.png.aspx;.pdf)
Figure 3: Dose distribution (top row) and clinical target volume motion trajectory (bottom row) for a single prostate radiotherapy fraction that was delivered with the MR-linac to our institute. The presented information was generated using our dose reconstruction workflow based on data routinely acquired during MR-guided radiotherapy. By processing 3D MR images, 2D cine MR images and treatment machine logfiles, we are able to assess the dosimetric impact of intrafractional anatomical changes. Figure taken from Menten et al. (2020), Radiotherapy and Oncology 145, pp. 88-94.
Can you comment on the impact of your work to the field?
When I started my PhD, there was still much uncertainty regarding whether it was possible to deliver radiotherapy treatments safely in the presence of a 1.5T magnetic field. Our work – among that of others – proved that accounting for the effects of the magnetic field during treatment planning enabled design of clinical acceptable treatments.
During the remainder of my PhD, we focused mostly on the development of strategies to implement and evaluate MR-guided real-time adaptive radiotherapy. For example, our systematic study of 2D cine MR imaging for MLC tracking showed that a single, low-resolution 2D MR image enabled 3D tumour localisation. Our dose reconstruction framework, which can be adopted easily by others, was also developed to quantify the dosimetric effects of intrafractional anatomical changes and to explore the benefit of real-time adaptive techniques. Ultimately, I hope that the findings of my PhD can help to make MR-guided MLC tracking a clinical reality.
What was the most challenging part during your PhD?
While the plans to install the MR-linac at the ICR were made in 2014, it took almost four years before the machine was commissioned and the first patient was treated. Large parts of the PhD proposal envisaged me conducting experiments with the MR-linac. As it became clear that this would not be possible, I had to replace these experiments with computer simulations, conduct them using clinical MR scanners or reinvent parts of my project by exploring other research ideas.
Who or what inspired you most during your studies?
It was very inspiring to work in a multidisciplinary team with many smart colleagues, both at my institute and as part of our research collaborations. I was able to learn continuously by talking to radiotherapy and MR physicists, computer scientists, medical staff and engineers.
When did you defend your thesis and who was your supervisor?
I defended my thesis in December 2018. In the United Kingdom, PhD theses are commonly assessed by two external experts – in my case Professor Marianne Aznar from the University of Manchester and Professor Tom Depuydt from KU Leuven. My supervisory team during my PhD studies consisted of my primary supervisor Professor Uwe Oelfke and associate supervisors Dr Simeon Nill and Dr Martin Fast. I am very grateful for all their advice, support and encouragement.
Will you stay in the field? What are your plans for the future?
I decided to switch research fields after having worked in radiotherapy research for more than eight years. As I very much enjoyed the programming and image processing tasks during my PhD, I looked to join a computer science department. In August 2019, I started a postdoctoral position in the BioMedIA group at Imperial College London. I am now working with Professor Daniel Rueckert on the development of machine learning methods for biomedical image analysis. My current research focuses on the use of deep learning to advance the understanding of age-related macular degeneration, the leading cause of blindness in the developed world. Still, I try to stay informed about the latest developments in radiotherapy. In the future, I can imagine returning to radiotherapy research and applying some of the techniques I am currently developing.
About the author
Martin Menten recently joined the BioMedIA group at Imperial College London as a postdoctoral researcher. Working together with Professor Daniel Rueckert, he is exploring the use of machine learning to advance the understanding and diagnosis of age-related macular degeneration, the leading cause of blindness in the elderly. Previously, he received his PhD in physics from the Institute of Cancer Research in London in 2018. As part of the team of Professor Uwe Oelfke, he researched strategies to adapt external beam radiotherapy to anatomical changes in real time in order to increase cure rates and to reduce side effects of cancer treatments. Before that, he obtained his Bachelor’s and Master’s degrees in physics at Heidelberg University in Germany, while completing research projects at the German Cancer Research Center and University of Wisconsin-Madison, USA.

Martin Menten
BioMedIA group
Imperial College London
London, United Kingdom
m.menten@imperial.ac.uk