ESTRO 2023 Physics Track - Big Data, Big Headache Session
How we tackle image segmentation in radiation oncology
My ‘headache’ was largely focused on the difficulty of image segmentation of the liver and colorectal liver metastasis while I was working on my PhD at MD Anderson Cancer Center at the University of Houston, Texas, USA. The difficulties could be boiled down to a few key challenges. First, contrast is often present within a CT to assist in the segmentation of contouring of colortecal liver mets. The amount of contrast that is present in the liver, the burden of disease, and the contrast in the surrounding tissues is highly dependant on both the patient and therapist administering the contrast. From a segmentation perspective, models perform at their best when images are presented consistently (you can imagine the difficulty as analogous to you trying to use a camera to take a photo of you beside the setting sun/when the sun is directly overhead. The contrast between you and the background will vary greatly between these two) Second, colortecal liver met tumour size and extent can vary dramatically. Some patients have single lesions that are very small and easily circumscribed, while other patients have large /multiple lesions throughout the liver. This presents a training issue with your deep learning model; you need a good balance of tumour and non-tumour. If the model is being trained to properly classify the lesion, and 99% of the liver is not lesion, the model can be lazy and simply say that there is no lesion present (and still be 99% right!).
For the first problem, regarding different levels of contrast in the liver for tumour segmentation, our group created a patient-specific histogram normalisation. Rather than applying a global normalisation, as we had previously to contour the entire liver, we focused on normalising specifically to the values present in the liver. The general idea was that in our colorectal-liver metastases, the disease tended to be hypo-enhancing compared with the normal liver.
For our second problem, regarding variations in disease burden, we split the liver into variable-sized cubes that contained both diseased and normal liver. Batches of these cubes were used to train the model, ensuring a balanced presentation of both classes. The final result of this process was a model capable of segmenting colorectal liver metastasis across a variety of contrast enhancements. The paper, which explains everything in more detail, is present here:
https://www.ncbi.nlm.nih.gov/pmc/articles/PMC9403767/ [ncbi.nlm.nih.gov].
This work was an essential part of our Phase 2 clinical trial of colorectal liver ablation in interventional radiology, which enabled computational deformation of the patient’s liver while in the surgical suite, and guidance regarding needle placement.
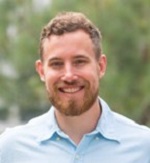
Brian Mark Anderson, PhD
Medical Physicist
Department of Radiation Oncology
University of North Carolina at Chapel Hill School of Medicine
Chapel Hill, North Carolina, USA